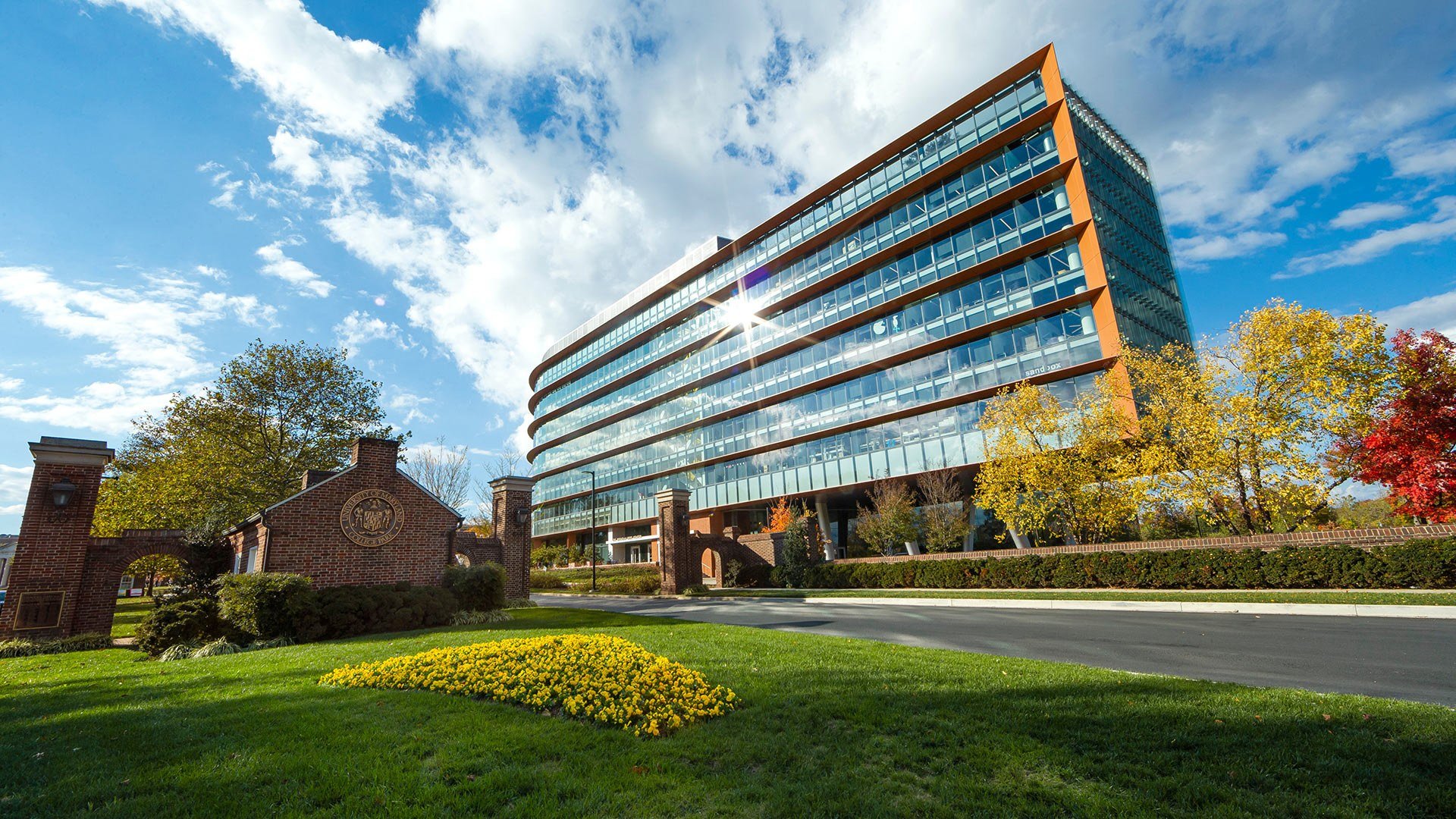
Wei Ai
Assistant Professor of Information
University of Maryland
Area of Expertise: Data Analysis For Social Good
Wei Ai is an assistant professor in the College of Information with an appointment in the University of Maryland Institute for Advanced Computer Studies. His research on large-scale behavior data analysis employs methodologies like machine learning, causal inference, and experimental design, with interests in social good, computational social science, recommender systems, and network analysis.
-
Ai, W., Chen, Y., Mei, Q., Ye, J., & Zhang, L. (2023). Putting Teams into the Gig Economy: A Field Experiment at a Ride-Sharing Platform. Management Science, 69(9), 5336–5353.
Abstract: The gig economy provides workers with the benefits of autonomy and flexibility but at the expense of work identity and coworker bonds. Among the many reasons why gig workers leave their platforms, one unexplored aspect is the lack of an organization identity. In this study, we develop a team formation and interteam contest field experiment at a ride-sharing platform. We assign drivers to teams either randomly or based on similarity in age, hometown location, or productivity. Having these teams compete for cash prizes, we find that (1) compared with those in the control condition, treated drivers work longer hours and earn 12% higher revenue during the contest; (2) the treatment effect persists two weeks postcontest, albeit with half of the effect size; and (3) drivers in hometown-similar teams are more likely to communicate with each other, whereas those in age-similar teams continue to work longer hours and earn higher revenue during the two weeks after the contest ends. Together, our results show that platform designers can leverage team identity and team contests to increase revenue and worker engagement in a gig economy.
-
Ye, T., Ai, W., Chen, Y., Mei, Q., Ye, J., & Zhang, L. (2022). Virtual teams in a gig economy. Proceedings of the National Academy of Sciences, 119(51).
Abstract: While the gig economy provides flexible jobs for millions of workers globally, a lack of organization identity and coworker bonds contributes to their low engagement and high attrition rates. To test the impact of virtual teams on worker productivity and retention, we conduct a field experiment with 27,790 drivers on a ride-sharing platform. We organize drivers into teams that are randomly assigned to receiving their team ranking, or individual ranking within their team, or individual performance information (control). We find that treated drivers work longer hours and generate significantly higher revenue. Furthermore, drivers in the team-ranking treatment continue to be more engaged 3 mo after the end of the experiment. A machine-learning analysis of 149 team contests in 86 cities suggests that social comparison, driver experience, and within-team similarity are the key predictors of the virtual team efficacy.
-
Ai, W., Chen, R., Chen, Y., Mei, Q., & Phillips, W. (2016). Recommending teams promotes prosocial lending in online microfinance. Proceedings of the National Academy of Sciences, 113(52), 14944–14948.
Abstract: This paper reports the results of a large-scale field experiment designed to test the hypothesis that group membership can increase participation and prosocial lending for an online crowdlending community, Kiva. The experiment uses variations on a simple email manipulation to encourage Kiva members to join a lending team, testing which types of team recommendation emails are most likely to get members to join teams as well as the subsequent impact on lending. We find that emails do increase the likelihood that a lender joins a team, and that joining a team increases lending in a short window (1 wk) following our intervention. The impact on lending is large relative to median lender lifetime loans. We also find that lenders are more likely to join teams recommended based on location similarity rather than team status. Our results suggest team recommendation can be an effective behavioral mechanism to increase prosocial lending.
-
Xu, P., Liu, J., Jones, N., Cohen, J., & Ai, W. (2024). The Promises and Pitfalls of Using Language Models to Measure Instruction Quality in Education. In Proceedings of the 2024 Conference of the North American Chapter of the Association for Computational Linguistics: Human Language Technologies (Volume 1: Long Papers).
Abstract: Assessing instruction quality is a fundamental component of any improvement efforts in the education system. However, traditional manual assessments are expensive, subjective, and heavily dependent on observers’ expertise and idiosyncratic factors, preventing teachers from getting timely and frequent feedback. Different from prior research that mostly focuses on low-inference instructional practices on a singular basis, this paper presents the first study that leverages Natural Language Processing (NLP) techniques to assess multiple high-inference instructional practices in two distinct educational settings: in-person K-12 classrooms and simulated performance tasks for pre-service teachers. This is also the first study that applies NLP to measure a teaching practice that is widely acknowledged to be particularly effective for students with special needs. We confront two challenges inherent in NLP-based instructional analysis, including noisy and long input data and highly skewed distributions of human ratings. Our results suggest that pretrained Language Models (PLMs) demonstrate performances comparable to the agreement level of human raters for variables that are more discrete and require lower inference, but their efficacy diminishes with more complex teaching practices. Interestingly, using only teachers’ utterances as input yields strong results for student-centered variables, alleviating common concerns over the difficulty of collecting and transcribing high-quality student speech data in in-person teaching settings. Our findings highlight both the potential and the limitations of current NLP techniques in the education domain, opening avenues for further exploration.
-
Zhou, Y., Lu, X., Gao, G., Mei, Q., & Ai, W. (2024). Emoji promotes developer participation and issue resolution on GitHub. Proceedings of the International AAAI Conference on Web and Social Media, 18, 1833–1846.
Abstract: Although remote working is increasingly adopted during the pandemic, many are concerned by the low-efficiency in the remote working. Missing in text-based communication are non-verbal cues such as facial expressions and body language, which hinders the effective communication and negatively impacts the work outcomes. Prevalent on social media platforms, emojis, as alternative non-verbal cues, are gaining popularity in the virtual workspaces well. In this paper, we study how emoji usage influences developer participation and issue resolution in virtual workspaces. To this end, we collect GitHub issues for a one-year period and apply causal inference techniques to measure the causal effect of emojis on the outcome of issues, controlling for confounders such as issue content, repository, and author information. We find that emojis can significantly reduce the resolution time of issues and attract more user participation. We also compare the heterogeneous effect on different types of issues. These findings deepen our understanding of the developer communities, and they provide design implications on how to facilitate interactions and broaden developer participation.