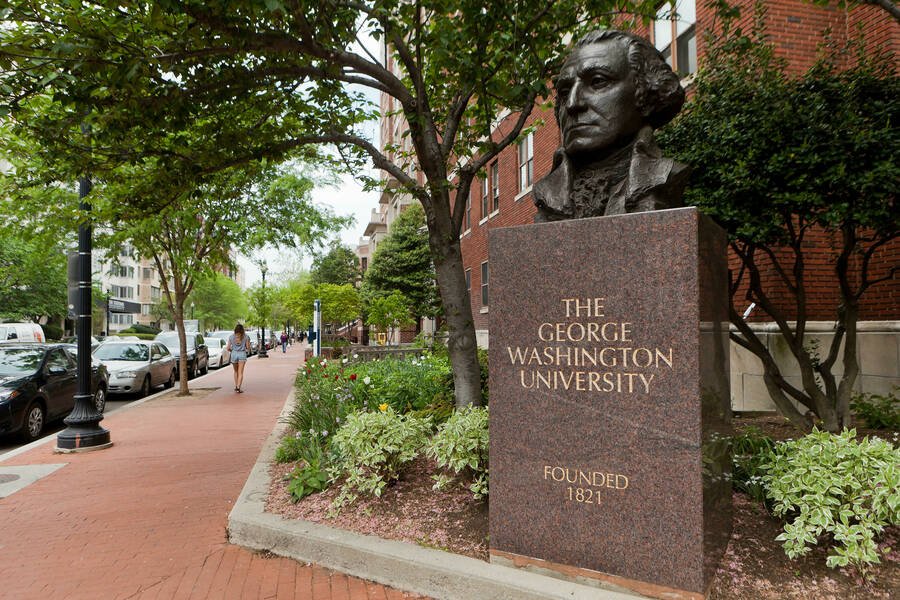
Peng Wei
Associate Professor of Mechanical and Aerospace Engineering
George Washington University
Area of Expertise: Trustworthy AI in Safety-Critical Systems
Peng Wei is an associate professor of mechanical and aerospace engineering at George Washington University where he leads the Intelligent Aerospace Systems Lab. By contributing to the intersection of control, optimization, machine learning, and artificial intelligence, Wei develops autonomy and human-in-the-loop decision making systems for aeronautics, aviation and aerial robotics.
-
Zhao, Z., Lee, J., Li, Z., Park, C. H., & Wei, P. (2023). Vision-based Perception with Safety Awareness for UAS Autonomous Landing. George Washington University.
Abstract: The use of small unmanned aircraft systems (UAS) has shown great potential for last-mile package delivery and medical supply transportation. In order to achieve higher levels of autonomy, scale up operations without tele-operating human pilots, and ensure landing safety during off-nominal events (e.g. people, pets, bikes, or cars being near/on the designated landing pad), we propose a robust, real-time deep learning based safe landing perception algorithm to (1) identify the landing pad, and (2) detect static or moving obstacles/humans near or on the landing pad. Specifically in this paper, we compare two state-of-art deep learning based computer vision models for object detection: RetinaNet and YOLOv5 to detect potential obstacles (pedestrians, cars and etc.). Additionally, we design and build a landing pad based on ArUco fiducial markers so we can detect the relative position and angle between the landing pad and the UAS with the ArUco library. Finally, we combined the landing pad and potential obstacle detection algorithms to ensure the landing pad is clear of obstacles. Our algorithm achieves real-time performance with 30 frame per second (FPS) video, suitable for real-world applications and further development.
-
Guo, W., Zhou, Y., & Wei, P. (2022). Exploring Online and Offline Explainability in Deep Reinforcement Learning for Aircraft Separation Assurance. Frontiers in Aerospace Engineering.
Abstract: Deep Reinforcement Learning (DRL) has demonstrated promising performance in maintaining safe separation among aircraft. In this work, we focus on a specific engineering application of aircraft separation assurance in structured airspace with high-density air traffic. In spite of the scalable performance, the non-transparent decision-making processes of DRL hinders human users from building trust in such learning-based decision making tool. In order to build a trustworthy DRL-based aircraft separation assurance system, we propose a novel framework to provide stepwise explanations of DRL policies for human users. Based on the different needs of human users, our framework integrates 1) a Soft Decision Tree (SDT) as an online explanation provider to display critical information for human operators in real-time; and 2) a saliency method, Linearly Estimated Gradient (LEG), as an offline explanation tool for certification agencies to conduct more comprehensive verification time or post-event analyses. Corresponding visualization methods are proposed to illustrate the information in the SDT and LEG efficiently: 1) Online explanations are visualized with tree plots and trajectory plots; 2) Offline explanations are visualized with saliency maps and position maps. In the BlueSky air traffic simulator, we evaluate the effectiveness of our framework on case studies with complex airspace route structures. Results show that the proposed framework can provide reasonable explanations of multi-agent sequential decision-making. In addition, for more predictable and trustworthy DRL models, we investigate two specific patterns that DRL policies follow based on similar aircraft locations in the airspace.
-
Baheri, A., Ren, H., Johnson, B., Razzaghi, P., & Wei, P. (2022). A Verification Framework for Certifying Learning-Based Safety-Critical Aviation Systems. George Washington University.
Abstract: We present a safety verification framework for design-time and run-time assurance of learning-based components in aviation systems. Our proposed framework integrates two novel methodologies. From the design-time assurance perspective, we propose offline mixed-fidelity verification tools that incorporate knowledge from different levels of granularity in simulated environments. From the run-time assurance perspective, we propose reachability- and statistics-based online monitoring and safety guards for a learning-based decision-making model to complement the offline verification methods. This framework is designed to be loosely coupled among modules, allowing the individual modules to be developed using independent methodologies and techniques, under varying circumstances and with different tool access. The proposed framework offers feasible solutions for meeting system safety requirements at different stages throughout the system development and deployment cycle, enabling the continuous learning and assessment of the system product.
-
Guo, W., Brittain, M., & Wei, P. (2022). Safety Enhancement for Deep Reinforcement Learning in Autonomous Separation Assurance. arXiv preprint arXiv:2105.02331.
Abstract: The separation assurance task will be extremely challenging for air traffic controllers in a complex and high-density airspace environment. Deep reinforcement learning (DRL) was used to develop an autonomous separation assurance framework in our previous work where the learned model advised speed maneuvers. In order to improve the safety of this model in unseen environments with uncertainties, in this work we propose a safety module for DRL in autonomous separation assurance applications. The proposed module directly addresses both model uncertainty and state uncertainty to improve safety. Our safety module consists of two sub-modules: (1) the state safety sub-module is based on the execution-time data augmentation method to introduce state disturbances in the model input state; (2) the model safety sub-module is a Monte-Carlo dropout extension that learns the posterior distribution of the DRL model policy. We demonstrate the effectiveness of the two sub-modules in an open-source air traffic simulator with challenging environment settings. Through extensive numerical experiments, our results show that the proposed sub-safety modules help the DRL agent significantly improve its safety performance in an autonomous separation assurance task.
-
Brittain, M. W., Yang, X., & Wei, P. (2020). A Deep Multi-Agent Reinforcement Learning Approach to Autonomous Separation Assurance. arXiv preprint arXiv:2003.08353.
Abstract: A novel deep multi-agent reinforcement learning framework is proposed to identify and resolve conflicts among a variable number of aircraft in a high-density, stochastic, and dynamic sector. Currently the sector capacity is constrained by human air traffic controller's cognitive limitation. We investigate the feasibility of a new concept (autonomous separation assurance) and a new approach to push the sector capacity above human cognitive limitation. We propose the concept of using distributed vehicle autonomy to ensure separation, instead of a centralized sector air traffic controller. Our proposed framework utilizes Proximal Policy Optimization (PPO) that we modify to incorporate an attention network. This allows the agents to have access to variable aircraft information in the sector in a scalable, efficient approach to achieve high traffic throughput under uncertainty. Agents are trained using a centralized learning, decentralized execution scheme where one neural network is learned and shared by all agents. The proposed framework is validated on three challenging case studies in the BlueSky air traffic control environment. Numerical results show the proposed framework significantly reduces offline training time, increases performance, and results in a more efficient policy.