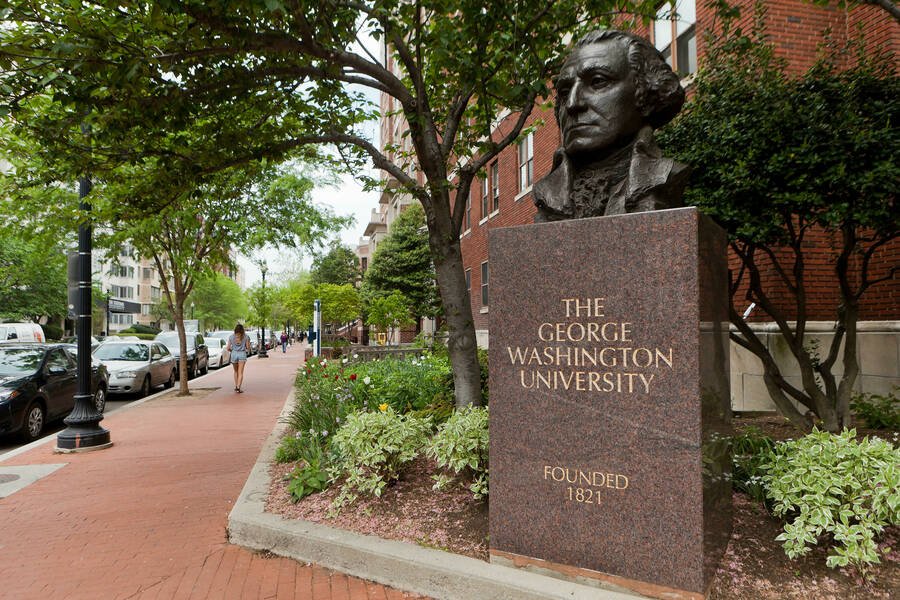
Chung Hyuk Park
Associate Professor of Biomedical Engineering
George Washington University
Chung Hyuk Park is an associate professor of biomedical engineering at George Washington University. His Assistive Robotics and Tele-Medicine Lab studies the collaborative innovation between human intelligence and robotic technology, integrating machine learning, computer vision, haptics, and telepresence robotics. Park’s research focuses on three main themes: multi-modal human-robot interaction and robotic assistance for individuals with disabilities or special needs, robotic learning and humanized intelligence, and tele-medical robotic assistance.
Area of Expertise: Human-Robot Interaction
-
Zhao, Z., Chung, E., Chung, K. M., & Park, C. H. (2024). AV-FOS: A Transformer-based Audio-Visual Interaction Style Recognition for Children with Autism based on the Family Observation Schedule (FOS-II). Authorea Preprints.
Abstract: Challenging behaviors in children with autism is a serious clinical condition, oftentimes leading to aggression or self-injurious actions The Family Observation Schedule 2nd Edition (FOS-II) is an intensive and finegrained scale used to observe and analyze the behaviors of individuals with autism, which facilitates the diagnosis and monitoring of autism severity. Previous AI-based approaches for automated behavior analysis in autism often focused on predicting facial expressions and body movements without generating a clinically meaningful scale, mostly utilizing visual information. In this study, we propose a deep-learning based algorithm with audiovisual multimodal-data clinically coded with the Family Observation Schedule 2nd Edition (FOS-II), named AV-FOS model. Our proposed AV-FOS model leverages transformer-based structure and self-supervised learning to intelligently recognize Interaction Styles (IS) in the FOS-II scale from subjects' video recordings. This enables the automatic generation of the FOS-II measures with clinically acceptable accuracy. We explore the IS recognition using a multimodal large language model, GPT4V, with prompt engineering provided with FOS-II measure definitions as the baseline for this study and compare with other vision-based deep learning algorithms. We believe this research represents a significant advancement in autism research and clinical accessibility. The proposed AV-FOS and our FOS-II dataset will serve as a gateway toward the digital health era for future AI models related to autism.
-
Sidulova, M., & Park, C. H. (2023). Conditional variational autoencoder for functional connectivity analysis of autism spectrum disorder functional magnetic resonance imaging data: a comparative study. Bioengineering, 10(10), 1209.
Abstract: Generative models, such as Variational Autoencoders (VAEs), are increasingly employed for atypical pattern detection in brain imaging. During training, these models learn to capture the underlying patterns within “normal” brain images and generate new samples from those patterns. Neurodivergent states can be observed by measuring the dissimilarity between the generated/reconstructed images and the input images. This paper leverages VAEs to conduct Functional Connectivity (FC) analysis from functional Magnetic Resonance Imaging (fMRI) scans of individuals with Autism Spectrum Disorder (ASD), aiming to uncover atypical interconnectivity between brain regions. In the first part of our study, we compare multiple VAE architectures—Conditional VAE, Recurrent VAE, and a hybrid of CNN parallel with RNN VAE—aiming to establish the effectiveness of VAEs in application FC analysis. Given the nature of the disorder, ASD exhibits a higher prevalence among males than females. Therefore, in the second part of this paper, we investigate if introducing phenotypic data could improve the performance of VAEs and, consequently, FC analysis. We compare our results with the findings from previous studies in the literature. The results showed that CNN-based VAE architecture is more effective for this application than the other models.
-
Xie, B., & Park, C. H. (2023). Multi-modal correlated network with emotional reasoning knowledge for social intelligence question-answering. In Proceedings of the IEEE/CVF International Conference on Computer Vision (pp. 3075-3081).
Abstract: The capacity for social reasoning is essential to the development of social intelligence in humans, which we easily acquire through study and experience. The acquisition of such ability by machines, however, is still challenging, even with the diverse deep learning models that are currently available. Recent artificial social intelligence models have achieved state-of-the-art results in question-answering tasks by employing a variety of methods, including self-supervised setups, multi-modal inputs, and so on. However, there is still a gap in the literature regarding the introduction of commonsense knowledge when training the model in social intelligence tasks. In this paper, we propose a Multi-Modal Temporal Correlated Network with Emotional Social Cues (MMTC-ESC). In order to model cross-modal correlations, an attention-based mechanism is used, and contrastive learning is achieved using emotional social cues. Our findings indicate that combining multimodal inputs and using contrastive loss is advantageous for the performance of social intelligence learning.
-
Xie, B., Milam, G., Ning, B., Cha, J., & Park, C. H. (2022). DXM‐TransFuse U-net: Dual cross-modal transformer fusion U-net for automated nerve identification. Computerized Medical Imaging and Graphics, 99, 102090.
Abstract: Accurate nerve identification is critical during surgical procedures to prevent damage to nerve tissues. Nerve injury can cause long-term adverse effects for patients, as well as financial overburden. Birefringence imaging is a noninvasive technique derived from polarized images that have successfully identified nerves that can assist during intraoperative surgery. Furthermore, birefringence images can be processed under 20 ms with a GPGPU implementation, making it a viable image modality option for real-time processing. In this study, we first comprehensively investigate the usage of birefringence images combined with deep learning, which can automatically detect nerves with gains upwards of 14% over its color image-based (RGB) counterparts on the F2 score. Additionally, we develop a deep learning network framework using the U-Net architecture with a Transformer based fusion module at the bottleneck that leverages both birefringence and RGB modalities. The dual-modality framework achieves 76.12 on the F2 score, a gain of 19.6 % over single-modality networks using only RGB images. By leveraging and extracting the feature maps of each modality independently and using each modality’s information for cross-modal interactions, we aim to provide a solution that would further increase the effectiveness of imaging systems for enabling noninvasive intraoperative nerve identification.
-
Javed, H., & Park, C. H. (2022). Promoting Social Engagement With a Multi-Role Dancing Robot for In-Home Autism Care. Frontiers in Robotics and AI, 9, 880691.
Abstract: This work describes the design of real-time dance-based interaction with a humanoid robot, where the robot seeks to promote physical activity in children by taking on multiple roles as a dance partner. It acts as a leader by initiating dances but can also act as a follower by mimicking a child’s dance movements. Dances in the leader role are produced by a sequence-to-sequence (S2S) Long Short-Term Memory (LSTM) network trained on children’s music videos taken from YouTube. On the other hand, a music orchestration platform is implemented to generate background music in the follower mode as the robot mimics the child’s poses. In doing so, we also incorporated the largely unexplored paradigm of learning-by-teaching by including multiple robot roles that allow the child to both learn from and teach to the robot. Our work is among the first to implement a largely autonomous, real-time full-body dance interaction with a bipedal humanoid robot that also explores the impact of the robot roles on child engagement. Importantly, we also incorporated in our design formal constructs taken from autism therapy, such as the least-to-most prompting hierarchy, reinforcements for positive behaviors, and a time delay to make behavioral observations. We implemented a multimodal child engagement model that encompasses both affective engagement (displayed through eye gaze focus and facial expressions) as well as task engagement (determined by the level of physical activity) to determine child engagement states. We then conducted a virtual exploratory user study to evaluate the impact of mixed robot roles on user engagement and found no statistically significant difference in the children’s engagement in single-role and multiple-role interactions. While the children were observed to respond positively to both robot behaviors, they preferred the music-driven leader role over the movement-driven follower role, a result that can partly be attributed to the virtual nature of the study. Our findings support the utility of such a platform in practicing physical activity but indicate that further research is necessary to fully explore the impact of each robot role.
-
Xie, B., Sidulova, M., & Park, C. H. (2021). Robust multimodal emotion recognition from conversation with transformer-based crossmodality fusion. Sensors, 21(14), 4913.
Abstract: Decades of scientific research have been conducted on developing and evaluating methods for automated emotion recognition. With exponentially growing technology, there is a wide range of emerging applications that require emotional state recognition of the user. This paper investigates a robust approach for multimodal emotion recognition during a conversation. Three separate models for audio, video and text modalities are structured and fine-tuned on the MELD. In this paper, a transformer-based crossmodality fusion with the EmbraceNet architecture is employed to estimate the emotion. The proposed multimodal network architecture can achieve up to 65% accuracy, which significantly surpasses any of the unimodal models. We provide multiple evaluation techniques applied to our work to show that our model is robust and can even outperform the state-of-the-art models on the MELD.
-
Lee, J., Zhang, X., Park, C. H., & Kim, M. J. (2021). Real-time teleoperation of magnetic force-driven microrobots with 3D haptic force feedback for micro-navigation and micro-transportation. IEEE Robotics and Automation Letters, 6(2), 1769-1776.
Abstract: Untethered mobile microrobots controlled by an external magnetic gradient field can be employed as advanced biomedical applications inside the human body such as cell therapy, micromanipulation, and noninvasive surgery. Haptic technology and telecommunication, on the other hand, can extend the potentials of untethered microrobot applications. In those applications, users can communicate with the robot operating system remotely to manipulate microrobots with haptic feedback. Haptic sensations artificially constructed by the wirelessly communicated information can assist human operators to experience forces while controlling the microrobots. The proposed system is composed of a haptic device and a magnetic tweezer system, both of which are integrated through a teleoperation technique based on network communication. Users can control the microrobots remotely and feel the haptic interactions with the remote environment in realtime. The 3D haptic environment is reconstructed dynamically by a model-free haptic rendering algorithm using a 2D planar image input of the microscope. The interaction between microrobots and environmental objects is haptically rendered as 3D objects to achieve spatial haptic operation with obstacle avoidance.Moreover, path generation and path guidance forces provide virtual interaction for human users to manipulate the microrobot by following the near-optimal path in path-following tasks. The potential applications of the presented system are medical remote treatment in different sites, remote drug delivery by avoiding physically penetrating through the skin, remotely-controlled cell manipulations, and biopsy without a biopsy needle.