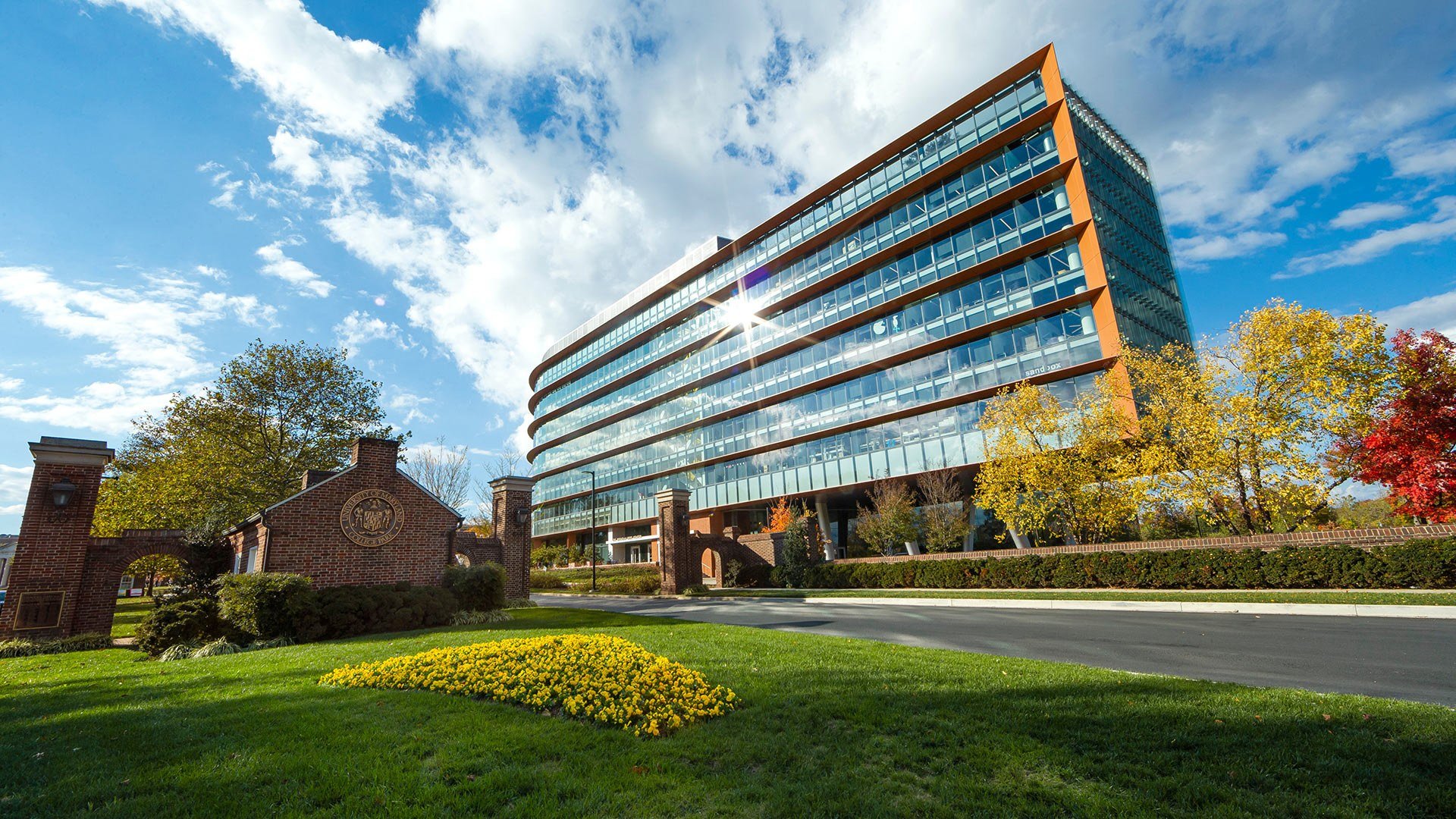
Pablo Paredes Castro
Assistant Professor of Computer Science
University of Maryland
Pablo Paredes Castro is an assistant professor of computer science with an appointment in the University of Maryland Institute for Advanced Computer Studies, where he also leads the Pervasive Wellbeing Tech Lab. His research focuses on subtle interventions to reduce stress, such as guiding people to breathe slowly with subtle haptic cues from office or car furniture, and passive sensing of affective and physiological biomarkers derived from existing devices (computers, phones, tablets, etc.).
Area of Expertise: AI for Mental Health
-
Tong, X., Mauriello, M. L., Mora-Mendoza, M. A., Prabhu, N., Kim, J. P., & Paredes, P. E. (2023). Just Do Something: Comparing Self-proposed and Machine-recommended Stress Interventions among Online Workers with Home Sweet Office. In Proceedings of the 2023 CHI Conference on Human Factors in Computing Systems (CHI '23), April 23–28, 2023, Hamburg, Germany. ACM, New York, NY, USA,.
Abstract: Modern stress management techniques have been shown to be effective, particularly when applied systematically and with the supervision of an instructor. However, online workers usually lack sufficient support from therapists and learning resources to self-manage their stress. To better assist these users, we implemented a browser-based application, Home Sweet Office (HSO), to administer a set of stress micro-interventions which mimic existing therapeutic techniques, including somatic, positive psychology, meta cognitive, and cognitive behavioral categories. In a four-week field study, we compared random and machine-recommended interventions to interventions that were self-proposed by participants in order to investigate effective content and recommendation methods. Our primary findings suggest that both machine-recommended and self-proposed interventions had significantly higher momentary efficacy than random selection, whereas machine-recommended interventions offer more activity diversity compared to self-proposed interventions. We conclude with reflections on these results, discuss features and mechanisms which might improve efficacy, and suggest areas for future work.
-
Mauriello, M. L., Tantivasadakarn, N., Mora-Mendoza, M. A., Lincoln, E. T., Hon, G., Nowruzi, P., Simon, D., Hansen, L., Goenawan, N. H., Kim, J., Gowda, N., Jurafsky, D., & Paredes, P. E. (2021). A Suite of Mobile Conversational Agents for Daily Stress Management (Popbots): Mixed Methods Exploratory Study. JMIR Formative Research, 5(9), e25294.
Abstract: Background: Approximately 60%-80% of the primary care visits have a psychological stress component, but only 3% of patients receive stress management advice during these visits. Given recent advances in natural language processing, there is renewed interest in mental health chatbots. Conversational agents that can understand a user's problems and deliver advice that mitigates the effects of daily stress could be an effective public health tool. However, such systems are complex to build and costly to develop. To address these challenges, our aim is to develop and evaluate a fully automated mobile suite of shallow chatbots—we call them Popbotst—that may serve as a new species of chatbots and further complement human assistance in an ecosystem of stress management support.
-
Kim, L. H., Saha, G., Leon, A. A., King, A. C., Mauriello, M. L., & Paredes, P. E. (2022). Shared Autonomy to Reduce Sedentary Behavior Among Sit-Stand Desk Users in the United States and India: Web-Based Study. JMIR Formative Research, 6(11).
Abstract: Fitness technologies such as wearables and sit-stand desks are increasingly being used to fight sedentary lifestyles by encouraging physical activity. However, adherence to such technologies decreases over time because of apathy and increased dismissal of behavioral nudges. To address this problem, we introduced shared autonomy in the context of sit-stand desks, where user input is integrated with robot autonomy to control the desk and reduce sedentary behavior and investigated user reactions and preferences for levels of automation with a sit-stand desk. As demographics affect user acceptance of robotic technology, we also studied how perceptions of non-volitional behavior change differ across cultures (United States and India), sex, familiarity, dispositional factors, and health priming messages.
-
Paredes, P., Gilad-Bachrach, R., Czerwinski, M., Roseway, A., Rowan, K., & Hernandez, J. (2014). PopTherapy: Coping with Stress through Pop-Culture. In Proceedings of the 8th International Conference on Pervasive Computing Technologies for Healthcare (PervasiveHealth '14). ICST (Institute for Computer Sciences, Social-Informatics and Telecommunications Engineering), 109–117.
Abstract: Stress is considered to be a modern day “global epidemic"; so given the widespread nature of this problem, it would be beneficial if solutions that help people to learn how to cope better with stress were scalable beyond what individual or group therapies can provide today. Therefore, in this work, we study the potential of smart-phones as a pervasive medium to provide therapy for the general population - "popular therapy". The work melds two novel contributions: first, a micro-intervention authoring process that focuses on repurposing popular web applications as stress management interventions; and second, a machine-learning based intervention recommender system that learns how to match interventions to individuals and their temporal circumstances over time. After four weeks, participants in our user study reported higher self-awareness of stress, lower depression-related symptoms and having learned new simple ways to deal with stress. Furthermore, participants receiving the machine-learning recommendations without option to select different ones showed a tendency towards using more constructive coping behaviors.
-
Matthew Louis Mauriello, Thierry Lincoln, Grace Hon, Dorien Simon, Dan Jurafsky, and Pablo Paredes. 2021. SAD: A Stress Annotated Dataset for Recognizing Everyday Stressors in SMS-like Conversational Systems. In Extended Abstracts of the 2021 CHI Conference on Human Factors in Computing Systems (CHI EA '21). Association for Computing Machinery, New York, NY, USA, Article 399, 1–7.
Abstract: There is limited infrastructure for providing stress management services to those in need. To address this problem, chatbots are viewed as a scalable solution. However, one limiting factor is having clear definitions and examples of daily stress on which to build models and methods for routing appropriate advice during conversations. We developed a dataset of 6850 SMS-like sentences that can be used to classify input using a scheme of 9 stressor categories derived from: stress management literature, live conversations from a prototype chatbot system, crowdsourcing, and targeted web scraping from an online repository. In addition to releasing this dataset, we show results that are promising for classification purposes. Our contributions include: (i) a categorization of daily stressors, (ii) a dataset of SMS-like sentences, (iii) an analysis of this dataset that demonstrates its potential efficacy, and (iv) a demonstration of its utility for implementation via a simulation of model response times.