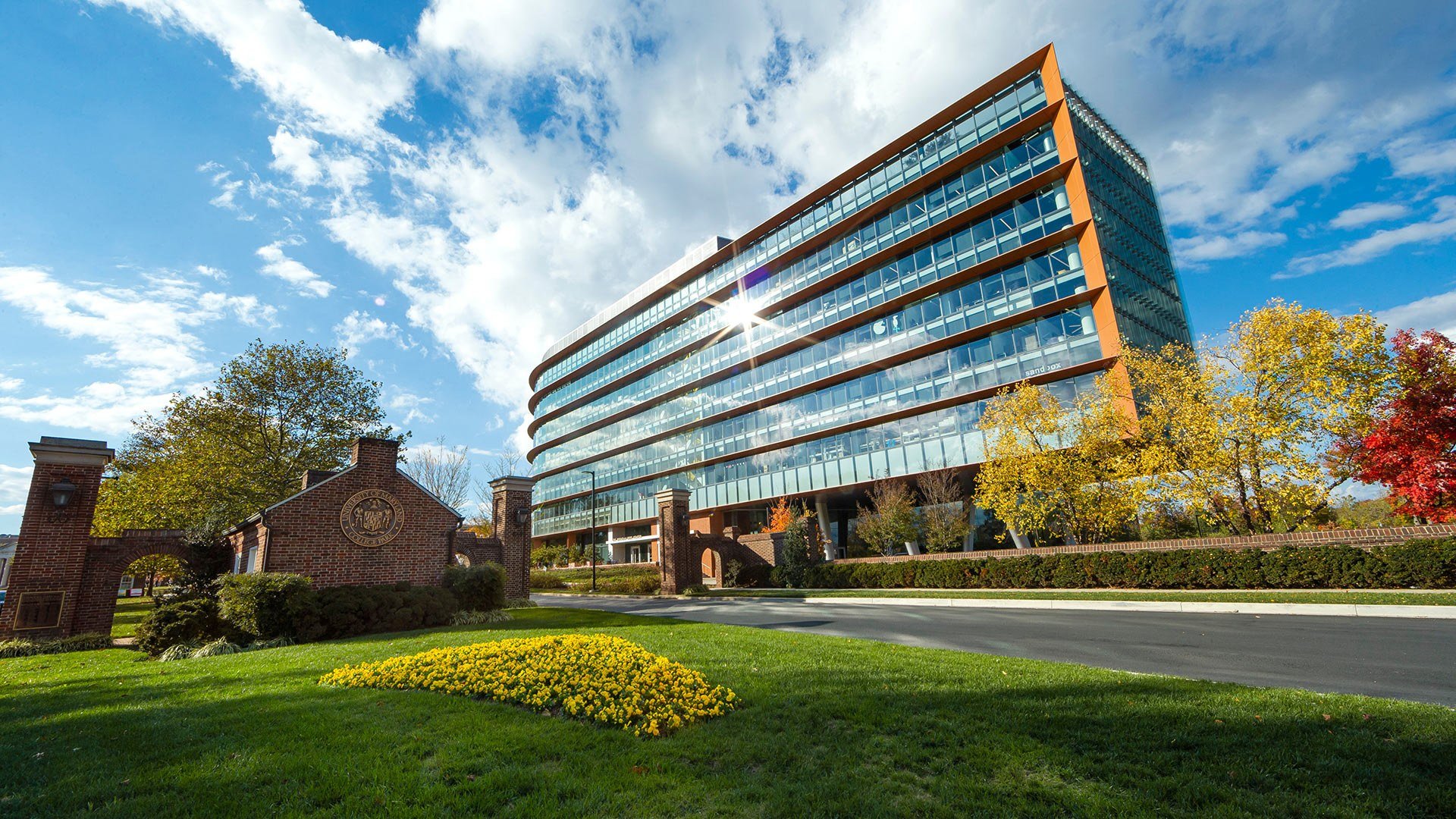
Ashwinee Panda
Postdoctoral Fellow, University of Maryland
Area of Expertise: Large Language Model Pretraining
Ashwinee Panda is a postdoctoral fellow at the Institute for Trustworthy AI in Law & Society (TRAILS) and the University of Maryland Institute for Advanced Computer Studies (UMIACS). He works on a number of topics in large language model pretraining. Panda is particularly interested in Mixture of Experts machine learning models, scaling laws, and scalable low-resource decentralized training.
-
McLeish, S., Kirchenbauer, J., Miller, D. Y., Singh, S., Bhatele, A., Goldblum, M., Panda, A., & Goldstein, T. (2025). Gemstones: A Model Suite for Multi-Faceted Scaling Laws. ArXiv (Cornell University).
Abstract: Scaling laws are typically fit using a family of models with a narrow range of frozen hyperparameter choices. In this work we study scaling laws using a wide range of architecture and hyperparameter choices, and highlight their impact on resulting prescriptions. As a primary artifact of our research, we release the Gemstones: the most comprehensive open-source scaling law dataset to date, consisting of over 4000 checkpoints from transformers with up to 2 billion parameters; these models have been trained with different learning rates, cooldown schedules, and architectural shapes. Our checkpoints enable more complex studies of scaling, such as a law that predicts language modeling performance as a function of model width and depth. By examining the various facets of our model suite, we find that the prescriptions of scaling laws can be highly sensitive to the experimental design process and the specific model checkpoints used during fitting.
-
Panda, A., Baherwani, V., Sarwar, Z., Thérien, B., Rawls, S., Sahu, S., Chakraborty, S., & Goldstein, T. (n.d.-b). Dense backpropagation improves routing for Sparsely-Gated Mixture-of-Experts. Workshop on Machine Learning and Compression, NeurIPS 2024, Vancouver, Canada
Abstract: Sparsely-gated Mixture-of-Experts (MoEs) have proven to be more effi2 cient than dense Transformers because they can dynamically activate a 3 subset of their overall parameters by routing tokens to selected “experts”, 4 allowing practitioners to scale up model parameter counts without sig5 nificantly increasing total compute. However, current MoE training ap6 proaches only update the router with a sparse gradient and suffer from 7 issues such as load imbalance. We propose a new router that can receive 8 a dense gradient update from a sparse forward pass. Our method adds 9 minimal overhead, but improves on the common Top-K routing in both 10 performance and load balance.
-
Jain, N., Shrivastava, A., Zhu, C., Liu, D., Samuel, A., Panda, A., Kumar, A., Goldblum, M., & Goldstein, T. (2024). Refusal tokens: a simple way to calibrate refusals in large language models. arXiv (Cornell University).
Abstract: A key component of building safe and reliable language models is enabling the models to appropriately refuse to follow certain instructions or answer certain questions. We may want models to output refusal messages for various categories of user queries, for example, ill-posed questions, instructions for committing illegal acts, or queries which require information past the model's knowledge horizon. Engineering models that refuse to answer such questions is complicated by the fact that an individual may want their model to exhibit varying levels of sensitivity for refusing queries of various categories, and different users may want different refusal rates. The current default approach involves training multiple models with varying proportions of refusal messages from each category to achieve the desired refusal rates, which is computationally expensive and may require training a new model to accommodate each user's desired preference over refusal rates. To address these challenges, we propose refusal tokens, one such token for each refusal category or a single refusal token, which are prepended to the model's responses during training. We then show how to increase or decrease the probability of generating the refusal token for each category during inference to steer the model's refusal behavior. Refusal tokens enable controlling a single model's refusal rates without the need of any further fine-tuning, but only by selectively intervening during generation.