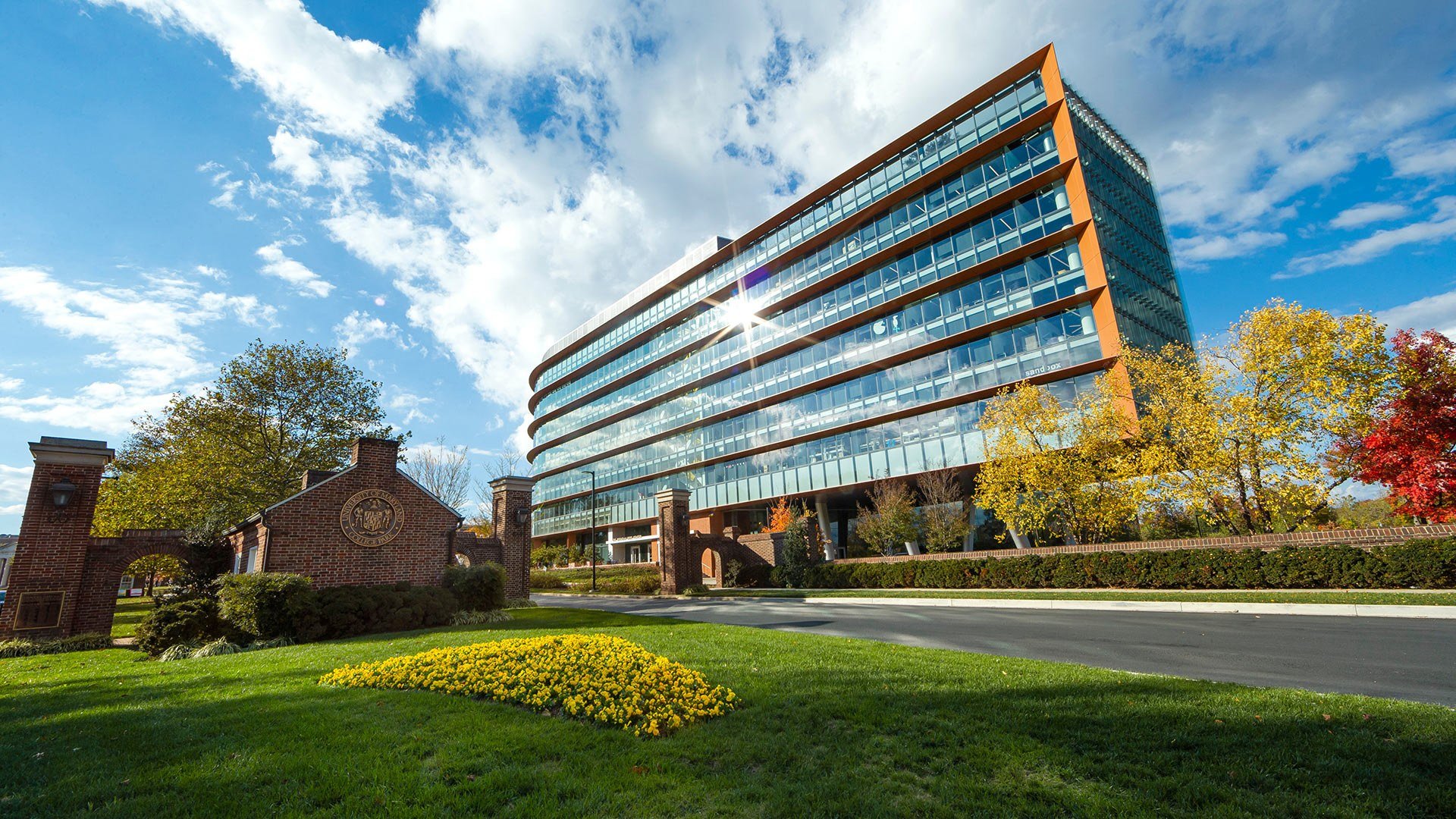
Michelle Mazurek
Associate Professor of Computer Science
University of Maryland
Areas of Expertise: Data, Bias, Privacy, and Targeted Advertising
Michelle Mazurek is an associate professor of computer science with an appointment in the University of Maryland Institute for Advanced Computer Studies. She also serves as director of the Maryland Cybersecurity Center and is a member of the Human-Computer Interaction Lab. Mazurek's research is focused on human elements of computer security and privacy. She specializes in understanding and supporting security- and privacy-related decision-making.
-
Luo, A. F., Greenstadt, R., Warford, N., Mazurek, M. L., Dooley, S., & McDonald, N. (n.d.). How Library IT Staff Navigate Privacy and Security Challenges and Responsibilities.
Abstract: Libraries provide critical IT services to patrons who lack access to computational and internet resources. We conducted 12 semi-structured interviews with library IT staff to learn about their privacy and security protocols and policies, the challenges they face implementing them, and how this relates to their patrons. We frame our findings using Sen’s capabilities approach and find that library IT staff are primarily concerned with protecting their patrons’ privacy from threats outside their walls—police, government authorities, and third parties. Despite their dedication to patron privacy, library IT staff frequently have to grapple with complex tradeoffs between providing easy, fluid, full-featured access to Internet technologies or third-party resources, protecting library infrastructure, and ensuring patron privacy.
-
Plane, A. C., Redmiles, E. M., Mazurek, M. L., & Tschantz, M. C. (2017). Exploring User Perceptions of Discrimination in Online Targeted Advertising. 935–951.
Abstract: Targeted online advertising now accounts for the largest share of the advertising market, beating out both TV and print ads. While targeted advertising can improve users’ online shopping experiences, it can also have negative effects. A plethora of recent work has found evidence that in some cases, ads may be discriminatory, leading certain groups of users to see better offers (e.g., job ads) based on personal characteristics such as gender. To develop policies around advertising and guide advertisers in making ethical decisions, one thing we must better understand is what concerns users and why. In an effort to answer this question, we conducted a pilot study and a multi-step main survey (n=2,086 in total) presenting users with different discriminatory advertising scenarios. We find that overall, 44% of respondents were moderately or very concerned by the scenarios we presented. Respondents found the scenarios significantly more problematic when discrimination took place as a result of explicit demographic targeting rather than in response to online behavior. However, our respondents’ opinions did not vary based on whether a human or an algorithm was responsible for the discrimination. These findings suggest that future policy documents should explicitly address discrimination in targeted advertising, no matter its origin, as a significant user concern, and that corporate responses that blame the algorithmic nature of the ad ecosystem may not be helpful for addressing public concerns.
-
Saha, D., Chan, A., Stacy, B., Javkar, K., Patkar, S., & Mazurek, M. L. (2020). User Attitudes On Direct-to-Consumer Genetic Testing. 2020 IEEE European Symposium on Security and Privacy (EuroS&P), 120–138.
Abstract: Advances in biotechnology now allow users to obtain their genetic information, including ancestry and predisposition to various diseases and health issues, with relative ease. With these new commercial services come a host of privacy concerns with respect to data sharing and access. User data is being sold to third parties, including pharmaceutical and biotechnology companies, and may be accessed by law enforcement in accordance with proper legal procedures. Moreover, many users of these services go on to deposit the data they obtain into online, public repositories that are fully accessible to anyone with an internet connection. The full extent of the risks they face may not be apparent to users. This paper reports on a semistructured interview study ( n=24 ) examining user concerns regarding these tests, what information they believe they are revealing, and what they think companies are doing with their data. We find that users are concerned with privacy, and understand at a basic level the nature of the data they are revealing. However, their privacy concerns are often insufficient to deter them from taking such a test, and many have difficulty grasping some of the implications of sharing their genetic information with commercial entities.
-
Saha, D., Schumann, C., Mcelfresh, D., Dickerson, J., Mazurek, M., & Tschantz, M. (2020). Measuring Non-Expert Comprehension of Machine Learning Fairness Metrics. Proceedings of the 37th International Conference on Machine Learning, 8377–8387.
Abstract: Bias in machine learning has manifested injustice in several areas, such as medicine, hiring, and criminal justice. In response, computer scientists have developed myriad definitions of fairness to correct this bias in fielded algorithms. While some definitions are based on established legal and ethical norms, others are largely mathematical. It is unclear whether the general public agrees with these fairness definitions, and perhaps more importantly, whether they understand these definitions. We take initial steps toward bridging this gap between ML researchers and the public, by addressing the question: does a lay audience understand a basic definition of ML fairness? We develop a metric to measure comprehension of three such definitions–demographic parity, equal opportunity, and equalized odds. We evaluate this metric using an online survey, and investigate the relationship between comprehension and sentiment, demographics, and the definition itself.
-
Vaidya, T., Votipka, D., Mazurek, M. L., & Sherr, M. (2019). Does Being Verified Make You More Credible? Account Verification’s Effect on Tweet Credibility. Proceedings of the 2019 CHI Conference on Human Factors in Computing Systems, 1–13.
Abstract: Many popular social networking and microblogging sites support verified accounts---user accounts that are deemed of public interest and whose owners have been authenticated by the site. Importantly, the content of messages contributed by verified account owners is not verified. Such messages may be factually correct, or not. This paper investigates whether users confuse authenticity with credibility by posing the question: Are users more likely to believe content from verified accounts than from non-verified accounts? We conduct two online studies, a year apart, with 748 and 2041 participants respectively, to assess how the presence or absence of verified account indicators influences users' perceptions of tweets. Surprisingly, across both studies, we find that---in the context of unfamiliar accounts---most users can effectively distinguish between authenticity and credibility. The presence or absence of an authenticity indicator has no significant effect on willingness to share a tweet or take action based on its contents.
-
Wei, M., Stamos, M., Veys, S., Reitinger, N., Goodman, J., Herman, M., Filipczuk, D., Weinshel, B., Mazurek, M. L., & Ur, B. (2020). What Twitter Knows: Characterizing Ad Targeting Practices, User Perceptions, and Ad Explanations Through Users’ Own Twitter Data. 145–162.
Abstract: Although targeted advertising has drawn significant attention from privacy researchers, many critical empirical questions remain. In particular, only a few of the dozens of targeting mechanisms used by major advertising platforms are well understood, and studies examining users’ perceptions of ad targeting often rely on hypothetical situations. Further, it is unclear how well existing transparency mechanisms, from data-access rights to ad explanations, actually serve the users they are intended for. To develop a deeper understanding of the current targeting advertising ecosystem, this paper engages 231 participants’ own Twitter data, containing ads they were shown and the associated targeting criteria, for measurement and user study. We find many targeting mechanisms ignored by prior work — including advertiser-uploaded lists of specific users, lookalike audiences, and retargeting campaigns — are widely used on Twitter. Crucially, participants found these understudied practices among the most privacy invasive. Participants also found ad explanations designed for this study more useful, more comprehensible, and overall more preferable than Twitter’s current ad explanations. Our findings underscore the benefits of data access, characterize unstudied facets of targeted advertising, and identify potential directions for improving transparency in targeted advertising.