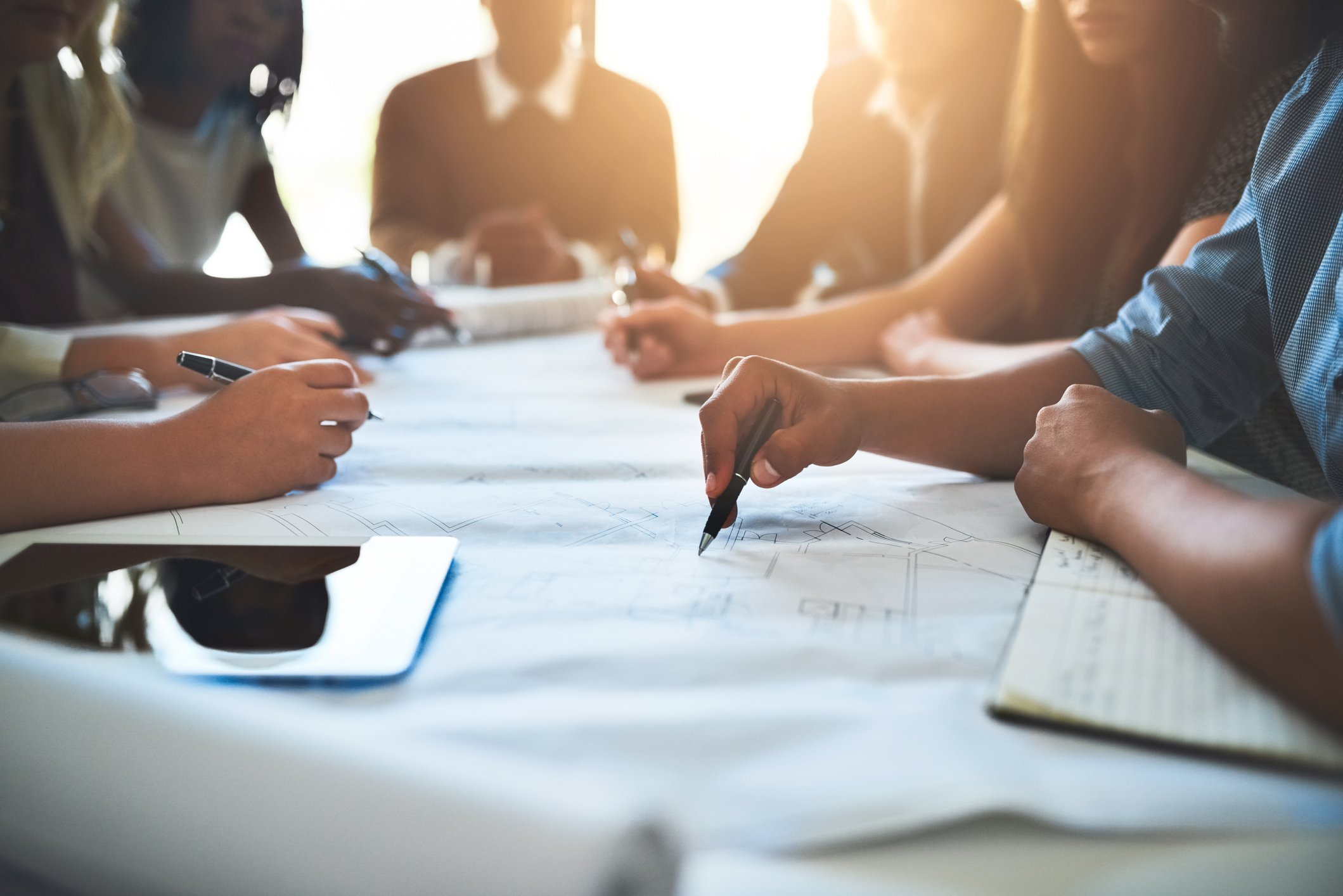
Founder and CEO of DataedX Group
Brandeis Marshall
Areas of Expertise: Algorithmic Bias, Ethics in Data Practices, Accountability, Transparency, Governance, and Race and Gender
Brandeis Marshall is the founder and CEO of DataedX Group, a data ethics learning and development agency for educators, scholars and practitioners to counteract automated oppression efforts with culturally-responsive instruction and strategies. Trained as a computer scientist and a former college professor, Marshall teaches, speaks and writes about the racial, gender, socioeconomic and socio-technical impact of data operations on technology and society.
-
Marshall, B. (2021). Algorithmic Misogynoir in Content Moderation Practice. Heinrich-Böll-Stiftung European Union and Heinrich-Böll-Stiftung.
Abstract: Existing content moderation practices, both algorithmically-driven and people-determined, are rooted in white colonialist culture. Black women’s opinions, experiences, and expertise are suppressed and their online communication streams are removed abruptly, silently, and quickly. Studying content moderation online has unearthed layers of algorithmic misogynoir, or racist misogyny directed against Black women. Tech companies, legislators and regulators in the U.S. have long ignored the continual mistreatment, misuse, and abuse of Black women online. This paper explores algorithmic misogynoir in content moderation and makes the case for the regular examination of the impact of content moderation tactics on Black women and other minoritized communities.
-
Marshall, B. (2022). Data Conscience: Algorithmic Siege on our Humanity. Wiley.
From the book’s back cover:
Data has enjoyed ‘bystander’ status as we’ve attempted to digitize responsibility and morality in tech. In fact, data’s importance should earn it a spot at the center of our thinking and strategy around building a better, more ethical world. It’s use―and misuse―lies at the heart of many of the racist, gendered, classist, and otherwise oppressive practices of modern tech.
In Data Conscience: Algorithmic Siege on our Humanity, computer science and data inclusivity thought leader Dr. Brandeis Hill Marshall delivers a call to action for rebel tech leaders, who acknowledge and are prepared to address the current limitations of software development. In the book, Dr. Brandeis Hill Marshall discusses how the philosophy of “move fast and break things” is, itself, broken, and requires change.
You’ll learn about the ways that discrimination rears its ugly head in the digital data space and how to address them with several known algorithms, including social network analysis, and linear regression.
-
Marshall, B., & Geier, S. (2019). Targeted Curricular Innovations in Data Science. 2019 IEEE Frontiers in Education Conference (FIE), 1–8.
Abstract: Many employers expect skills such as proper data generation, collection, storage, and analysis; however, these skills are often not taught in the undergraduate experience. Many STEM disciplines require computing coursework that includes coding in a modern programming language but does not explicitly address data stewardship. In this research, we present a faculty-focused data science program to address this educational gap. The faculty at two undergraduate institutions participate in a year-long slate of activities to learn about data science and design mechanisms for classroom dissemination. We describe the program details and provide sample classroom implementations as well as a summary of faculty post-participation perceptions.
-
Marshall, B., & Geier, S. (2020). Cross-Disciplinary Faculty Development in Data Science Principles for Classroom Integration. Proceedings of the 51st ACM Technical Symposium on Computer Science Education, 1207–1213.
Abstract: Data science in practice leverages the expertise in computer science, mathematics and statistics with applications in any field using data. The formalization of data science educational and pedagogical strategic remain in their infancy. College faculty from various disciplines are tasked with designing and delivering data science instruction without the formal knowledge of how data science principles are executed in practice. We call this the data science instruction gap. Also, these faculties are implementing their discipline's standard pedagogical strategies to their understanding of data science. In this paper, we present our cross-disciplinary instructional program model designed to narrow the data science instruction gap for faculty. It is designed to scaffold college faculties' data science learning to support their discipline-specific data science instruction. We provide individualized and group-based support structures to instill data science principles and transition them from learners to educators in data science. Lastly, we share our model's impact on and value to faculty as well as make recommendations for model adoption.
-
Monroe-White, T., Marshall, B., & Contreras-Palacios, D. H. (2022). Social Exclusion in Data Science: A Critical Exploration of Disparate Representation in Higher Education. AMCIS 2022 Proceedings.
Abstract: Information systems anchors itself as a bridge between the information technology professionals who support technological operations and the users that leverage these tools for their own productivity end-goals. As a society, we adjust to technological innovations at different rates based on our level of exposure to and investment in continued learning. However, how we learn and what we learn are highly dependent on who we are as learners. By examining the intersectional race/ethnicity and gender representation within the higher education infrastructure and applying the individual differences theory of social inclusion to national datasets of graduate students in computer and information sciences, mathematics and statistics we create a disparate representation metric for data science-related fields. Findings suggest intersectional variation in access to the broader information economy via systematic patterns of exclusion across institutions of higher education. Finally, recommendations are suggested to curb this marginalization.
-
Monroe-White, T., Marshall, B., & Contreras-Palacios, H. (2021). Waking up to Marginalization: Public Value Failures in Artificial Intelligence and Data Science. Proceedings of 2nd Workshop on Diversity in Artificial Intelligence (AIDBEI), 7–21.
Abstract: Data science education is increasingly becoming an integral part of many educational structures, both informal and formal. Much of the attention has been on the application of AI principles and techniques, especially machine learning, natural language processing and predictive analytics. While AI is only one phase in the data science ecosystem, we must embrace a fuller range of job roles that help manage AI algorithms and systems — from the AI innovators and architects (in CS, Math and Statistics) to the AI technicians and specialists (in CS, IT and IS). Also, it’s important that we better understand the current state of the low participation and representation of minoritized groups that further stifles the accessibility and inclusion efforts. However, how we learn and what we learn is highly dependent on who we are as learners. In this paper, we examine demographic disparities by race/ethnicity and gender within the information systems educational infrastructure from an evaluative perspective. More specifically, we adopt intersectional methods and apply the theory of public value failure to identify learning gaps in the fast-growing field of data science. National datasets of Master’s and Doctoral graduate students in IS, CS, Math and Statistics are used to create an “institutional parity score” which calculates field-specific representation by race/ethnicity and gender in data science related fields. We conclude by showcasing bias creep including the situational exclusion of individuals from access to the broader information economy, be it access to technologies and data or access to participate in the data workforce or data enabled-economic activity. Policy recommendations are suggested to curb and reduce this marginalization within information systems and related disciplines.