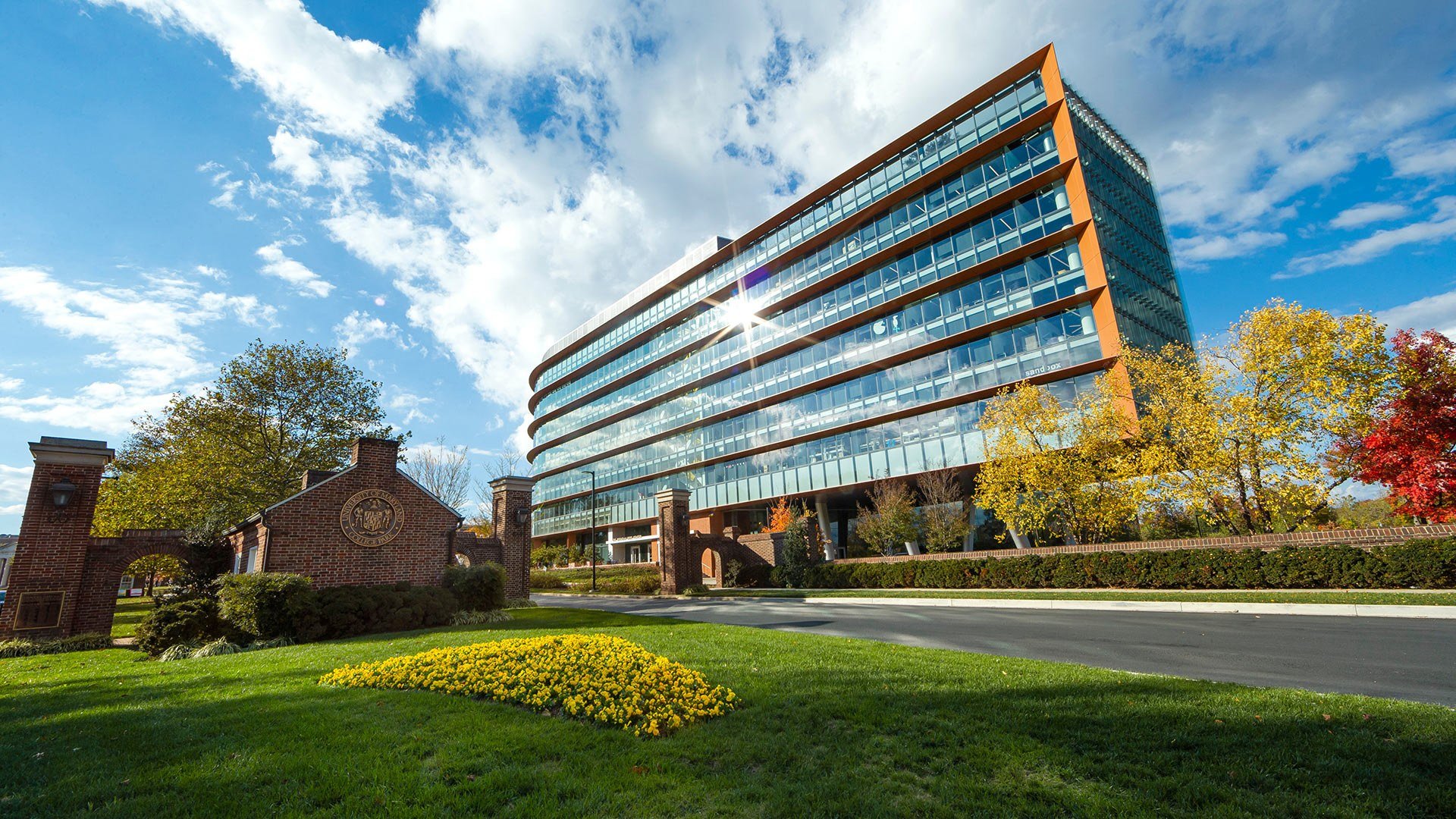
Hernisa Kacorri
Associate Professor of Information
University of Maryland
Hernisa Kacorri is an associate professor in the College of Information with an appointment in the the University of Maryland Institute for Advanced Computer Studies. She also holds affiliate appointments in the Department of Computer Science and the Human-Computer Interaction Lab, and serves as a core faculty member in the Trace Research and Development Center. The goal of Kacorri’s research is to build technologies that address real-world problems by integrating data-driven methods and human-computer interaction.
Area of Expertise: Accessible Design
-
Bragg, D., Koller, O., Bellard, M., Berke, L., Boudreault, P., Braffort, A., Caselli, N., Huenerfauth, M., Kacorri, H., Verhoef, T., Vogler, C., & Ringel Morris, M. (2019). Sign Language Recognition, Generation, and Translation: An Interdisciplinary Perspective. Proceedings of the 21st International ACM SIGACCESS Conference on Computers and Accessibility, 16–31.
Abstract: Developing successful sign language recognition, generation, and translation systems requires expertise in a wide range of fields, including computer vision, computer graphics, natural language processing, human-computer interaction, linguistics, and Deaf culture. Despite the need for deep interdisciplinary knowledge, existing research occurs in separate disciplinary silos, and tackles separate portions of the sign language processing pipeline. This leads to three key questions: 1) What does an interdisciplinary view of the current landscape reveal? 2) What are the biggest challenges facing the feld? and 3) What are the calls to action for people working in the feld? To help answer these questions, we brought together a diverse group of experts for a two-day workshop. This paper presents the results of that interdisciplinary workshop, providing key background that is often overlooked by computer scientists, a review of the state-of-the-art, a set of pressing challenges, and a call to action for the research community.
-
Dwivedi, U., Gandhi, J., Parikh, R., Coenraad, M., Bonsignore, E., & Kacorri, H. (2021). Exploring Machine Teaching with Children. 2021 IEEE Symposium on Visual Languages and Human-Centric Computing (VL/HCC), 1–11.
Abstract: Iteratively building and testing machine learning models can help children develop creativity, flexibility, and comfort with machine learning and artificial intelligence. We explore how children use machine teaching interfaces with a team of 14 children (aged 7-13 years) and adult co-designers. Children trained image classifiers and tested each other’s models for robustness. Our study illuminates how children reason about ML concepts, offering these insights for designing machine teaching experiences for children: (i) ML metrics (e.g. confidence scores) should be visible for experimentation; (ii) ML activities should enable children to exchange models for promoting reflection and pattern recognition; and (iii) the interface should allow quick data inspection (e.g. images vs. gestures).
-
Hong, J., Gandhi, J., Mensah, E. E., Zeraati, F. Z., Jarjue, E., Lee, K., & Kacorri, H. (2022). Blind Users Accessing Their Training Images in Teachable Object Recognizers. Proceedings of the 24th International ACM SIGACCESS Conference on Computers and Accessibility, 1–18.
Abstract: Teachable object recognizers provide a solution for a very practical need for blind people – instance level object recognition. They assume one can visually inspect the photos they provide for training, a critical and inaccessible step for those who are blind. In this work, we engineer data descriptors that address this challenge. They indicate in real time whether the object in the photo is cropped or too small, a hand is included, the photos is blurred, and how much photos vary from each other. Our descriptors are built into open source testbed iOS app, called MYCam. In a remote user study in (A=12) blind participants’ homes, we show how descriptors, even when error-prone, support experimentation and have a positive impact in the quality of training set that can translate to model performance though this gain is not uniform. Participants found the app simple to use indicating that they could effectively train it and that the descriptors were useful. However, many found the training being tedious, opening discussions around the need for balance between information, time, and cognitive load.
-
Hong, J., Lee, K., Xu, J., & Kacorri, H. (2020). Crowdsourcing the Perception of Machine Teaching. Proceedings of the 2020 CHI Conference on Human Factors in Computing Systems, 1–14.
Abstract: Teachable interfaces can empower end-users to attune machine learning systems to their idiosyncratic characteristics and environment by explicitly providing pertinent training examples. While facilitating control, their effectiveness can be hindered by the lack of expertise or misconceptions. We investigate how users may conceptualize, experience, and reflect on their engagement in machine teaching by deploying a mobile teachable testbed in Amazon Mechanical Turk. Using a performance-based payment scheme, Mechanical Turkers (N = 100) are called to train, test, and re-train a robust recognition model in real-time with a few snapshots taken in their environment. We find that participants incorporate diversity in their examples drawing from parallels to how humans recognize objects independent of size, viewpoint, location, and illumination. Many of their misconceptions relate to consistency and model capabilities for reasoning. With limited variation and edge cases in testing, the majority of them do not change strategies on a second training attempt.
-
Kamikubo, R., Lee, K., & Kacorri, H. (2023). Contributing to Accessibility Datasets: Reflections on Sharing Study Data by Blind People. Proceedings of the 2023 CHI Conference on Human Factors in Computing Systems, 1–18.
Abstract: To ensure that AI-infused systems work for disabled people, we need to bring accessibility datasets sourced from this community in the development lifecycle. However, there are many ethical and privacy concerns limiting greater data inclusion, making such datasets not readily available. We present a pair of studies where 13 blind participants engage in data capturing activities and reflect with and without probing on various factors that influence their decision to share their data via an AI dataset. We see how different factors influence blind participants’ willingness to share study data as they assess risk-benefit trade offs. The majority support sharing of their data to improve technology but also express concerns over commercial use, associated metadata, and the lack of transparency about the impact of their data. These insights have implications for the development of responsible practices for stewarding accessibility datasets, and can contribute to broader discussions in this area.
-
Kamikubo, R., Wang, L., Marte, C., Mahmood, A., & Kacorri, H. (2022). Data Representativeness in Accessibility Datasets: A Meta-Analysis. Proceedings of the 24th International ACM SIGACCESS Conference on Computers and Accessibility, 1–15.
Abstract: As data-driven systems are increasingly deployed at scale, ethical concerns have arisen around unfair and discriminatory outcomes for historically marginalized groups that are underrepresented in training data. In response, work around AI fairness and inclusion has called for datasets that are representative of various demographic groups. In this paper, we contribute an analysis of the representativeness of age, gender, and race & ethnicity in accessibility datasets–datasets sourced from people with disabilities and older adults—that can potentially play an important role in mitigating bias for inclusive AI-infused applications. We examine the current state of representation within datasets sourced by people with disabilities by reviewing publicly-available information of 190 datasets, we call these accessibility datasets. We find that accessibility datasets represent diverse ages, but have gender and race representation gaps. Additionally, we investigate how the sensitive and complex nature of demographic variables makes classification difficult and inconsistent (e.g., gender, race & ethnicity), with the source of labeling often unknown. By reflecting on the current challenges and opportunities for representation of disabled data contributors, we hope our effort expands the space of possibility for greater inclusion of marginalized communities in AI-infused systems.
-
Ozmen Garibay, O., Winslow, B., Andolina, S., Antona, M., Bodenschatz, A., Coursaris, C., Falco, G., Fiore, S. M., Garibay, I., Grieman, K., Havens, J. C., Jirotka, M., Kacorri, H., Karwowski, W., Kider, J., Konstan, J., Koon, S., Lopez-Gonzalez, M., Maifeld-Carucci, I., … Xu, W. (2023). Six Human-Centered Artificial Intelligence Grand Challenges. International Journal of Human–Computer Interaction, 39(3), 391–437.
Abstract: Widespread adoption of artificial intelligence (AI) technologies is substantially affecting the human condition in ways that are not yet well understood. Negative unintended consequences abound including the perpetuation and exacerbation of societal inequalities and divisions via algorithmic decision making. We present six grand challenges for the scientific community to create AI technologies that are human-centered, that is, ethical, fair, and enhance the human condition. These grand challenges are the result of an international collaboration across academia, industry and government and represent the consensus views of a group of 26 experts in the field of human-centered artificial intelligence (HCAI). In essence, these challenges advocate for a human-centered approach to AI that (1) is centered in human wellbeing, (2) is designed responsibly, (3) respects privacy, (4) follows human-centered design principles, (5) is subject to appropriate governance and oversight, and (6) interacts with individuals while respecting human’s cognitive capacities. We hope that these challenges and their associated research directions serve as a call for action to conduct research and development in AI that serves as a force multiplier towards more fair, equitable and sustainable societies.