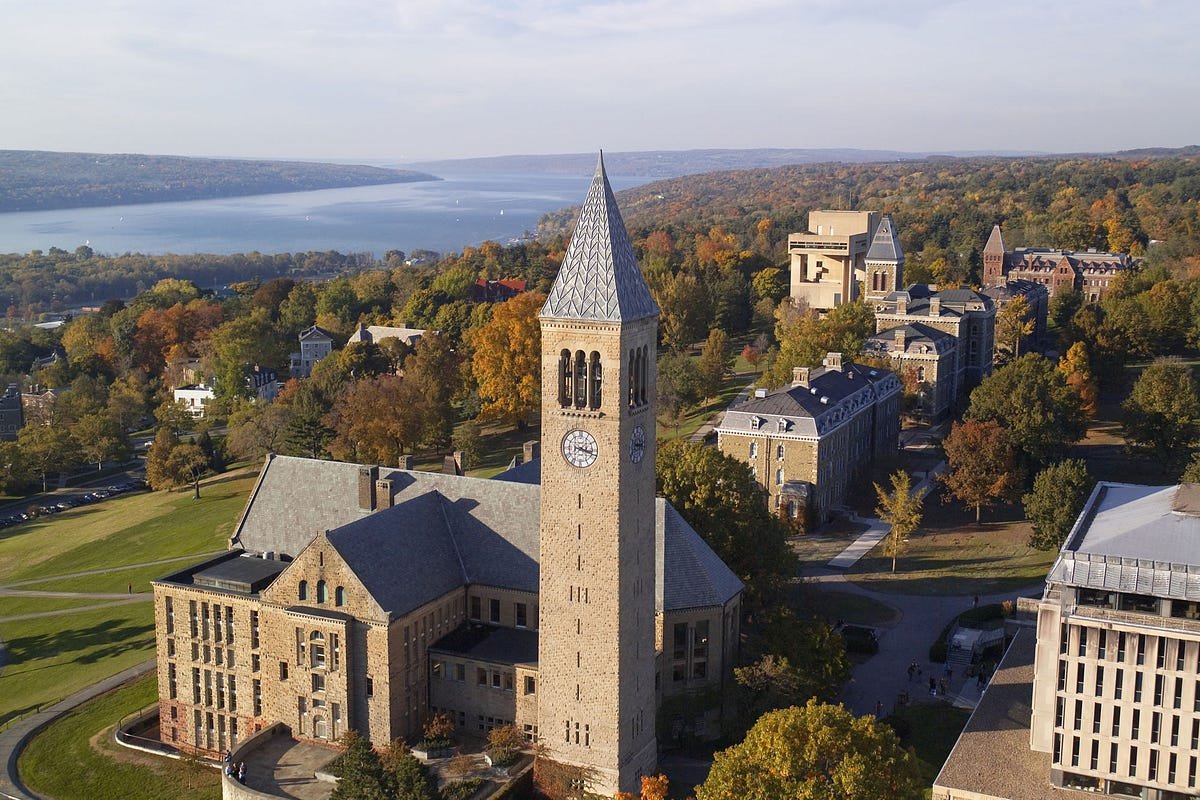
Malte Jung
Associate Professor of Information Science
Cornell University
Malte Jung is an associate professor of information science at Cornell University. His research seeks to build understanding about interpersonal dynamics in groups and teams and how those can be shaped by machines. Jung leads the Robots in Groups Lab, which focuses on design and behavioral aspects of human-robot interaction in group and team settings.
Area of Expertise: Human-robot Interaction
-
Sarah Strohkorb Sebo, Margaret Traeger, Malte Jung, and Brian Scassellati. 2018. The Ripple Effects of Vulnerability: The Effects of a Robot's Vulnerable Behavior on Trust in Human-Robot Teams. In Proceedings of the 2018 ACM/IEEE International Conference on Human-Robot Interaction (HRI '18). Association for Computing Machinery, New York, NY, USA, 178–186.
Abstract: Successful teams are characterized by high levels of trust between team members, allowing the team to learn from mistakes, take risks, and entertain diverse ideas. We investigated a robot's potential to shape trust within a team through the robot's expressions of vulnerability. We conducted a between-subjects experiment (N = 35 teams, 105 participants) comparing the behavior of three human teammates collaborating with either a social robot making vulnerable statements or with a social robot making neutral statements. We found that, in a group with a robot making vulnerable statements, participants responded more to the robot's comments and directed more of their gaze to the robot, displaying a higher level of engagement with the robot. Additionally, we discovered that during times of tension, human teammates in a group with a robot making vulnerable statements were more likely to explain their failure to the group, console team members who had made mistakes, and laugh together, all actions that reduce the amount of tension experienced by the team. These results suggest that a robot's vulnerable behavior can have "ripple effects" on their human team members' expressions of trust-related behavior.
-
Andrea Cuadra, Maria Wang, Lynn Andrea Stein, Malte F. Jung, Nicola Dell, Deborah Estrin, and James A. Landay. 2024. The Illusion of Empathy? Notes on Displays of Emotion in Human-Computer Interaction. In Proceedings of the CHI Conference on Human Factors in Computing Systems (CHI '24). Association for Computing Machinery, New York, NY, USA, Article 446, 1–18.
Abstract: From ELIZA to Alexa, Conversational Agents (CAs) have been deliberately designed to elicit or project empathy. Although empathy can help technology better serve human needs, it can also be deceptive and potentially exploitative. In this work, we characterize empathy in interactions with CAs, highlighting the importance of distinguishing evocations of empathy between two humans from ones between a human and a CA. To this end, we systematically prompt CAs backed by large language models (LLMs) to display empathy while conversing with, or about, 65 distinct human identities, and also compare how different LLMs display or model empathy. We find that CAs make value judgments about certain identities, and can be encouraging of identities related to harmful ideologies (e.g., Nazism and xenophobia). Moreover, a computational approach to understanding empathy reveals that despite their ability to display empathy, CAs do poorly when interpreting and exploring a user’s experience, contrasting with their human counterparts.
-
Ewart J. de Visser, Marieke M. M. Peeters, Malte F. Jung, Spencer Kohn, Tyler H. Shaw, Richard Pak, Mark A. Neerincx. Towards a Theory of Longitudinal Trust Calibration in Human–Robot Teams. International Journal of Social Robotics 12, 459–478 (2020).
Abstract: The introduction of artificial teammates in the form of autonomous social robots, with fewer social abilities compared to humans, presents new challenges for human–robot team dynamics. A key characteristic of high performing human-only teams is their ability to establish, develop, and calibrate trust over long periods of time, making the establishment of longitudinal human–robot team trust calibration a crucial part of these challenges. This paper presents a novel integrative model that takes a longitudinal perspective on trust development and calibration in human–robot teams. A key new proposed factor in this model is the introduction of the concept relationship equity. Relationship equity is an emotional resource that predicts the degree of goodwill between two actors. Relationship equity can help predict the future health of a long-term relationship. Our model is descriptive of current trust dynamics, predictive of the impact on trust of interactions within a human–robot team, and prescriptive with respect to the types of interventions and transparency methods promoting trust calibration. We describe the interplay between team trust dynamics and the establishment of work agreements that guide and improve human–robot collaboration. Furthermore, we introduce methods for dampening (reducing overtrust) and repairing (reducing undertrust) mis-calibrated trust between team members as well as methods for transparency and explanation. We conclude with a description of the implications of our model and a research agenda to jump-start a new comprehensive research program in this area.