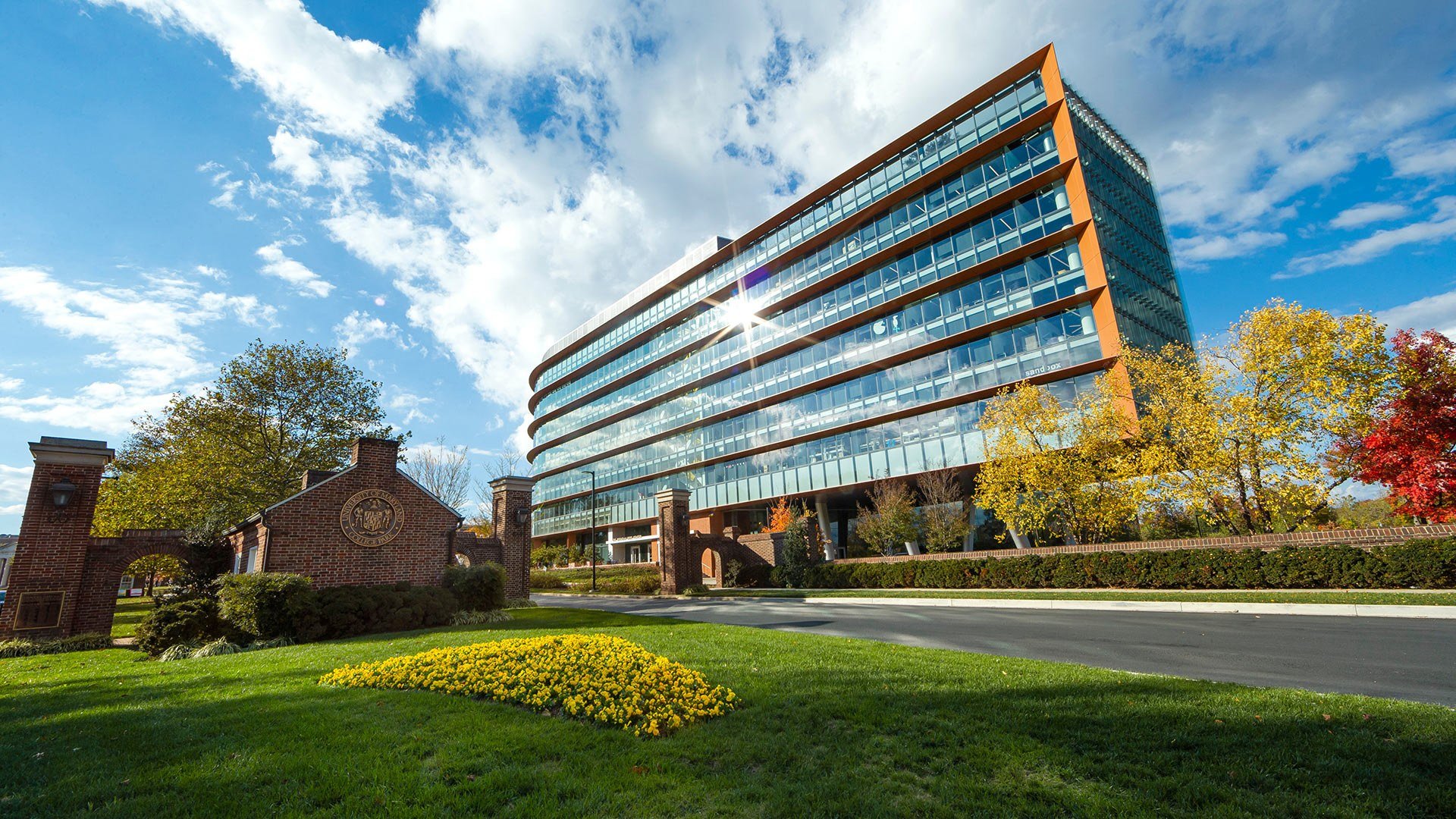
Zubin Jelveh
Assistant Professor of Information and Criminology and Criminal Justice
University of Maryland
Area of Expertise: Data Science for Public Policy
Zubin Jelveh is an assistant professor in the College of Information and the Department of Criminology and Criminal Justice at the University of Maryland. An expert in data science for public policy, record linkage, and the science of science, his research connects techniques from machine learning to problems in the social sciences.
-
Jelveh, Z., Kogut, B., & Naidu, S. (2024). The Economic Journal, 1–31.
Abstract: Does academic writing in economics reflect the political orientation of economists? We use machine learning to measure partisanship in academic economics articles. We predict the observed political behaviour of a subset of economists using phrases from their academic articles, show good out-of-sample predictive accuracy and then predict partisanship for all economists. We then use these predictions to examine patterns of political language in economics. We estimate journal-specific effects on predicted ideology, controlling for author and year fixed effects, that accord with existing survey-based measures. We show considerable sorting of economists into fields of research by predicted partisanship. We also show that partisanship is detectable even within fields, even across those estimating the same theoretical parameter. Using policy-relevant parameters collected from previous meta-analyses, we then show that imputed partisanship is correlated with estimated parameters, such that the implied policy prescription is consistent with partisan leaning. For example, we find that going from the most left-wing authored estimate of the taxable top income elasticity to the most right-wing authored estimate decreases the optimal tax rate from 77% to 60%.
-
Tahamont, S., Jelveh, Z., Chalfin, A., Yan, S., & Hansen, B. (2020). Journal of Quantitative Criminology, 37(3), 715–749.
Abstract: The increasing availability of large administrative datasets has led to an exciting innovation in criminal justice research—using administrative data to measure experimental outcomes in lieu of costly primary data collection. We demonstrate that this type of randomized experiment can have an unfortunate consequence: the destruction of statistical power. Combining experimental data with administrative records to track outcomes of interest typically requires linking datasets without a common identifer. In order to minimize mistaken linkages, researchers often use stringent linking rules like “exact matching” to ensure that speculative matches do not lead to errors in an analytic dataset. We show that this, seemingly conservative, approach leads to underpowered experiments, leaves real treatment effects undetected, and can therefore have profound implications for entire experimental literatures.
-
Guzman, J., Jelveh, Z., & Kogut, B. (n.d.). (2024).
Abstract: The norms of transparency and rigor are central to the institution of open science, incentivizing scientists to compete to produce knowledge and yet adhere to Mertonian norms (Merton, 1973). Nowhere are these norms more critical than in clinical trials, where the development of new medical interventions directly impacts human lives. In this paper, we first present evidence that professional norms in science have not prevented questionable research practices in academic clinical trials. Analyzing 10,072 primary p-values, we find little evidence of p-hacking among industry trials, a domain where private gains serve as the overarching incentive. We cannot, however, reject the hypothesis that p-hacking is prevalent in academic trials, which comprise 22% of the sample. We exploit the impact of an institutional intervention aimed at promoting Mertonian norms labeled SPIRIT 2013. Spirit is a set of guidelines endorsed by leading journals aimed at improving the creation of clinical trial study protocols. We find trials initiated after SPIRIT 2013 exhibited lower levels of p-hacking. Linguistic analysis shows that trial registrations more closely adhering to SPIRIT guidelines had a lower incidence of p-hacking. Additionally, in a dataset of over 300 thousand medical publications, only those relied upon clinical trials demonstrated a reduction in phacking following the introduction of SPIRIT 2013. Finally, while SPIRIT 2013 appears to reduce the incidence of p-hacking, it does not eliminate it entirely. Our findings underscore the relevance of institutional interventions to improving the quality of scientific findings, particularly in the critical domain of medical research.
-
Heller, S. B., Jakubowski, B., Jelveh, Z., & Kapustin, M. (2022). National Bureau of Economic Research.
Abstract: This paper shows that shootings are predictable enough to be preventable. Using arrest and victimization records for almost 644,000 people from the Chicago Police Department, we train a machine learning model to predict the risk of being shot in the next 18 months. Out-of-sample accuracy is strikingly high: of the 500 people with the highest predicted risk, almost 13 percent are shot within 18 months, a rate 128 times higher than the average Chicagoan. A central concern is that algorithms may “bake in” bias found in police data, overestimating risk for people likelier to interact with police conditional on their behavior. We show that Black male victims more often have enough police contact to generate predictions. But those predictions are not, on average, inflated; the demographic composition of predicted and actual shooting victims is almost identical. There are legal, ethical, and practical barriers to using these predictions to target law enforcement. But using them to target social services could have enormous preventive benefits: predictive accuracy among the top 500 people justifies spending up to $134,400 per person for an intervention that could cut the probability of being shot by half.
-
Arora, A., Beberman, X., Jelveh, Z., & Motta, A. (2024).
Abstract: Domestic violence accounts for 50% of female homicides in the U.S. The criminal justice system – with which the majority of victims initiate contact in the years leading up to their deaths – may be uniquely suited to prevent these tragedies. There remains considerable debate, however, on whether victims at high risk are identifiable, and whether criminal justice system responses targeted towards them can be effective. We study this approach in Chicago, where victims gauged to beat highest risk are selected for additional outreach, prosecutorial, and advocacy resources to increase the likelihood of successful criminal prosecution. Leveraging variation in prosecutors’ tendencies to classify cases as high risk, we show that this approach rapidly and persistently lowers the likelihood of homicide for victims on the margin of inclusion. Additionally, prosecutors are proficient at identifying high-risk victims, considerably outperforming standard machine learning algorithms.