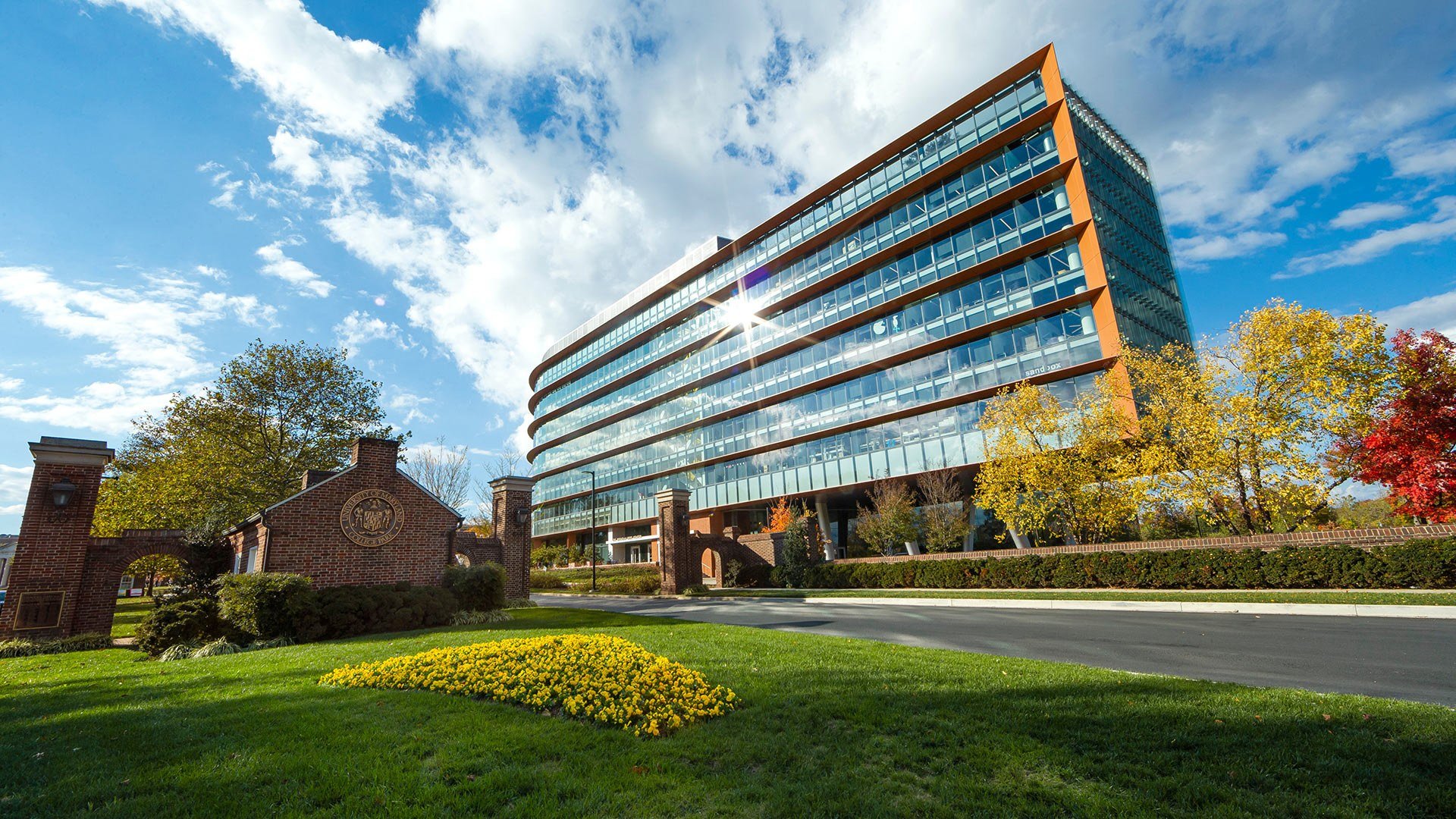
Naeemul Hassan
Assistant Professor of Journalism and Information University of Maryland
Naeemul Hassan is an assistant professor in the Philip Merrill College of Journalism and the College of Information at the University of Maryland where he also directs the Computational Journalism Lab. Hassan’s research interests include computational journalism, social media sensing, data mining, and natural language processing.
Area of Expertise: Quality of Information
-
Afsana, F., Kabir, M. A., Hassan, N., & Paul, M. (2021). Automatically Assessing Quality of Online Health Articles. IEEE Journal of Biomedical and Health Informatics, 25(2), 591–601.
Abstract: Today, information in the world wide web is overwhelmed by unprecedented quantity of data on versatile topics with varied quality. However, the quality of information disseminated in the field of medicine has been questioned as the negative health consequences of health misinformation can be life-threatening. There is currently no generic automated tool for evaluating the quality of online health information spanned over broad range. To address this gap, in this paper, we applied data mining approach to automatically assess the quality of online health articles based on 10 quality criteria. We have prepared a labelled dataset with 53012 features and applied different feature selection methods to identify the best feature subset with which our trained classifier achieved an accuracy of 84%−90% varied over 10 criteria. Our semantic analysis of features shows the underpinning associations between the selected features & assessment criteria and further rationalize our assessment approach. Our findings will help in identifying high quality health articles and thus aiding users in shaping their opinion to make right choice while picking health related help from online.
-
Ali, M., & Hassan, N. (2022). A Survey of Computational Framing Analysis Approaches. Proceedings of the 2022 Conference on Empirical Methods in Natural Language Processing, 9335–9348.
Abstract: Framing analysis is predominantly qualitative and quantitative, examining a small dataset with manual coding. Easy access to digital data in the last two decades prompts scholars in both computation and social sciences to utilize various computational methods to explore frames in large-scale datasets. The growing scholarship, however, lacks a comprehensive understanding and resources of computational framing analysis methods. Aiming to address the gap, this article surveys existing computational framing analysis approaches and puts them together. The research is expected to help scholars and journalists gain a deeper understanding of how frames are being explored computationally, better equip them to analyze frames in large-scale datasets, and, finally, work on advancing methodological approaches.
-
D. Molina, M., Sundar, S. S., Rony, M. M. U., Hassan, N., Le, T., & Lee, D. (2021). Does Clickbait Actually Attract More Clicks? Three Clickbait Studies You Must Read. Proceedings of the 2021 CHI Conference on Human Factors in Computing Systems, 1–19.
Abstract: Studies show that users do not reliably click more often on headlines classified as clickbait by automated classifiers. Is this because the linguistic criteria (e.g., use of lists or questions) emphasized by the classifiers are not psychologically relevant in attracting interest, or because their classifications are confounded by other unknown factors associated with assumptions of the classifiers? We address these possibilities with three studies—a quasi-experiment using headlines classified as clickbait by three machine-learning models (Study 1), a controlled experiment varying the headline of an identical news story to contain only one clickbait characteristic (Study 2), and a computational analysis of four classifiers using real-world sharing data (Study 3). Studies 1 and 2 revealed that clickbait did not generate more curiosity than non-clickbait. Study 3 revealed that while some headlines generate more engagement, the detectors agreed on a classification only 47% of the time, raising fundamental questions about their validity.
-
Hassan, N., Arslan, F., Li, C., & Tremayne, M. (2017). Toward Automated Fact-Checking: Detecting Check-worthy Factual Claims by ClaimBuster. Proceedings of the 23rd ACM SIGKDD International Conference on Knowledge Discovery and Data Mining, 1803–1812.
Abstract: This paper introduces how ClaimBuster, a fact-checking platform, uses natural language processing and supervised learning to detect important factual claims in political discourses. The claim spotting model is built using a human-labeled dataset of check-worthy factual claims from the U.S. general election debate transcripts. The paper explains the architecture and the components of the system and the evaluation of the model. It presents a case study of how ClaimBuster live covers the 2016 U.S. presidential election debates and monitors social media and Australian Hansard for factual claims. It also describes the current status and the long-term goals of ClaimBuster as we keep developing and expanding it.
-
Hassan, N., Yousuf, M., Mahfuzul Haque, M., A. Suarez Rivas, J., & Khadimul Islam, M. (2019). Examining the Roles of Automation, Crowds and Professionals Towards Sustainable Fact-checking. Companion Proceedings of The 2019 World Wide Web Conference, 1001–1006.
Abstract: This study explores an online fact-checking community called political fact-checking on reddit.com that relies on crowdsourcing to find and verify check-worthy facts relating to U.S. politics. The community embodies a network journalism model in which the process of finding and verifying check-worthy facts through crowdsourcing is coordinated by a team of moderators. Applying the concepts of connective journalism, this study analyzed the posts (N = 543) and comments (N = 10, 221) on the community’s Reddit page to understand differences in roles of the community members and the moderators. A mixed-method approach was used to analyze the data. The authors also developed an automated argument classification model to analyze the contents and identify ways to automate parts of the process. The findings suggest that a model consisting of crowds, professionals, and computer-assisted analysis could increase efficiency and decrease costs in news organizations that involve fact-checking.
-
Mahfuzul Haque, M., Yousuf, M., Shatil Alam, A., Saha, P., Ishtiaque Ahmed, S., & Hassan, N. (2020). Combating Misinformation in Bangladesh: Roles and Responsibilities as Perceived by Journalists, Fact-checkers, and Users. In ArXiv e-prints.
Abstract: There has been a growing interest within CSCW community in understanding the characteristics of misinformation propagated through computational media, and the devising techniques to address the associated challenges. However, most work in this area has been concentrated on the cases in the western world leaving a major portion of this problem unaddressed that is situated in the Global South. This paper aims to broaden the scope of this discourse by focusing on this problem in the context of Bangladesh, a country in the Global South. The spread of misinformation on Facebook in Bangladesh, a country with a population over 163 million, has resulted in chaos, hate attacks, and killings. By interviewing journalists, fact-checkers, in addition to surveying the general public, we analyzed the current state of verifying misinformation in Bangladesh. Our findings show that most people in the `news audience' want the news media to verify the authenticity of online information that they see online. However, the newspaper journalists say that fact-checking online information is not a part of their job, and it is also beyond their capacity given the amount of information being published online everyday. We further find that the voluntary fact-checkers in Bangladesh are not equipped with sufficient infrastructural support to fill in this gap. We show how our findings are connected to some of the core concerns of CSCW community around social media, collaboration, infrastructural politics, and information inequality. From our analysis, we also suggest several pathways to increase the impact of fact-checking efforts through collaboration, technology design, and infrastructure development.