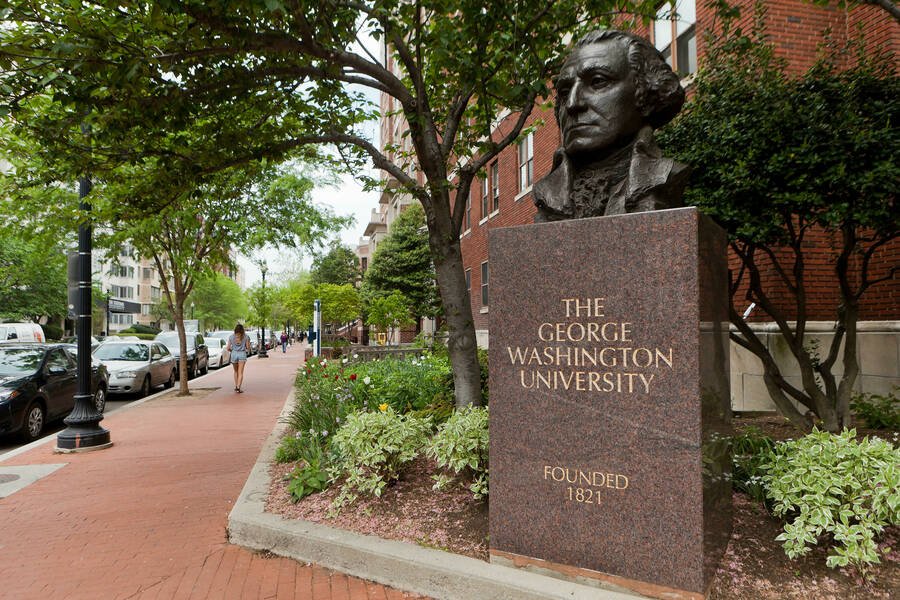
Erica Gralla
Associate Professor of Engineering Management and Systems Engineering
George Washington University
Area of Expertise: Optimizing Complex Systems
Erica Gralla is an associate professor in the Department of Engineering Management and Systems Engineering at George Washington University. She studies operations and supply chain management in disaster response and other urgent or uncertain environments. Gralla’s research seeks to combine the strengths of human intuition and mathematical models to create better decision-making approaches.
-
Gralla, E., & Goentzel, J. (2018). Humanitarian Transportation Planning: Evaluation of Practice-Based Heuristics and Recommendations for Improvement. European Journal of Operational Research.
Abstract: Transportation bottlenecks are a common and critical problem in humanitarian response. There is a need for better planning and prioritization of vehicles to transport humanitarian aid to affected communities. Optimization approaches have been developed for transportation planning, but adoption has been limited, due in part to the difficulty of implementation. This paper develops the basis for an easily implementable decision support tool by building on current planning practices in the humanitarian sector. We draw on an observational study to describe current planning practices, then develop heuristic algorithms that represent the observed planning processes, and compare their solutions to each other and to those of a mixed-integer linear program. We identify key weaknesses to guide the development of more sophisticated heuristics or optimization models that fit with current planning practices. We also find that a simple practice-driven heuristic performs well when it prioritizes deliveries based on destination priority or distance, and we argue that automating such a heuristic in a decision support tool would maintain the simplicity and transparency to enable implementation in practice, and improve planning by saving time and increasing accuracy and consistency.
-
Gralla, E., Goentzel, J., & Fine, C. (2016). Problem Formulation and Solution Mechanisms: A Behavioral Study of Humanitarian Transportation Planning. Production and Operations Management, 25(1), pp. 22-35.
Abstract: When dealing with urgent, ill-defined problems, such as rapidly evolving emergency situations, operations managers have little time for problem formulation or solution.While the mechanisms by which humans formulate and solve problems have been described, mechanisms for rapid, concurrent formulating and solving are not well under-stood. This paper investigates these mechanisms through a field study of transportation planning in a humanitarian response setting.The findings show that the problem is solved through greedy search and formulated through sensemaking, in which search enables updates to an evolving problem formulation, and the formulation directs and limits the search process. The paper explores the implications of these findings for the development of better problem formulation processes and problem-solving strategies for urgent and ill-defined operations management problems.
-
Gralla, E., Goentzel, J., & Fine, C. (2014). Assessing Trade-offs among Multiple Objectives for Humanitarian Aid Delivery Using Expert Preferences. Production and Operations Management, 23(6), pp. 978-989.
Abstract: Humanitarian aid agencies deliver emergency supplies and services to people affected by disasters. Scholars and practitioners have developed modeling approaches to support aid delivery planning, but they have used objective functions with little validation as to the trade-offs among the multiple goals of aid delivery. We develop a method to value the performance of aid delivery plans based on expert preferences over five key attributes: the amount of cargo delivered, the prioritization of aid by commodity type, the prioritization of aid by delivery location, the speed of delivery, and the operational cost. Through a conjoint analysis survey, we measure the preferences of 18 experienced humanitarian logisticians. The survey results quantify the importance of each attribute and enable the development of a piecewise linear utility function that can be used as an objective function in optimization models. The results show that the amount of cargo delivered is the most valued objective and cost the least important. In addition, experts prioritize more vulnerable communities and more critical commodities, but not to the exclusion of others. With these insights and the experts’ utility functions, better humanitarian objective functions can be developed to enable better aid delivery in emergency response.
-
C. Blair, E. Gralla, F. Wetmore, J. Goentzel, and M. Peters (2021). “A Systems Framework for International Development: The Data-Layered Causal Loop Diagram.” Production and Operations Management 30 (12).
Abstract: Meeting the United Nations Sustainable Development Goals (SDGs) will require adapting or redirecting a variety of very complex global and local human systems. It is essential that development scholars and practitioners have tools to understand the dynamics of these systems and the key drivers of their behavior, such as barriers to progress and leverage points for driving sustainable change. System dynamics tools are well suited to address this challenge, but they must first be adapted for the data-poor and fragmented environment of development work. Our key contribution is to extend the causal loop diagram (CLD) with a data layer that describes the status of and change in each variable based on available data. By testing dynamic hypotheses against the system's actual behavior, it enables analysis of a system's dynamics and behavioral drivers without simulation. The data-layered CLD was developed through a 4-year engagement with USAID/Uganda. Its contributions are illustrated through an application to agricultural financing in Uganda. Our analysis identified a lack of demand for agricultural loans as a major barrier to broadening agricultural financing, partially refuting an existing hypothesis that access to credit was the main constraint. Our work extends system dynamics theory to meet the challenges of this practice environment, enabling analysis of the complex dynamics that are crucial to achieving the SDGs.
-
J. Moline, J. Goentzel, and E. Gralla (2019). “Approaches for Locating and Staffing FEMA’s Disaster Recovery Centers.” Decision Sciences Journal 50(5), pp. 917-947.
Abstract: Disaster response is a challenging environment for data-driven decision support, because of its rarity, uniqueness, and high stakes. This article develops and demonstrates practical decision support approaches for an important problem faced by the U.S. Federal Emergency Management Agency (FEMA), identifies challenges and successes during their partial implementation, and explores how such approaches can encourage the adoption of data-driven decision-making in crisis situations. Specifically, this article develops approaches for locating and staffing temporary Disaster Recovery Centers (DRCs), which provide in-person service to disaster-affected communities. Working with FEMA, we developed two models that aim to improve service to survivors and minimize costs. One model fits easily into FEMA's current decision-making process, while the other further improves service by challenging some extant assumptions. By testing the models using data from three past disasters, we find that this decision support can result in cost savings of 75% on average by eliminating unnecessary DRCs and over-staffing and, at the same time, maintain or reduce the maximum travel time required for the disaster-affected population to access DRCs. Aspects of the models have already been used during disaster operations. FEMA's experience highlights the potential for data- and model-driven analyses to improve resource allocation and demonstrates an approach to improve organizational decisions by developing models that either align with or challenge the decision-making culture.
-
E. Gralla, J. Herrmann and M. Morency (2018). “Design Problem Decomposition: An Empirical Study of Small Teams of Facility Designers.” Research in Engineering Design.
Abstract: Decomposing complex design problems is an important component of design processes. When a design problem is too complex to solve all at once, the problem is decomposed into manageable subproblems. Previous work on design processes has identified some general decomposition patterns and has studied how individual designers decompose design problems; this study examines the way variables are grouped into subproblems, the process of decomposition, and whether small teams use similar decomposition patterns. Data were collected from five teams as they solved a facility design problem, and the subproblems that they considered were analyzed and compared. Using a mix of qualitative and quantitative analysis techniques, we examined (1) whether their subproblems group tightly coupled design variables (and separate weakly coupled variables); (2) whether their decompositions (subproblems and the sequence in which they were solved) follow a top–down design process; and (3) whether different teams used the same decompositions. Our results suggest that teams followed a partial top–down design process that moved from breadth- to depth-first search, and that subproblems were often driven by two types of coupling among design variables. However, the inconsistency of observed approaches suggests that there is room for improvement in how human designers decompose problems. By identifying these issues, the results lay a foundation for future research to provide better support for human designers in decomposing problems.