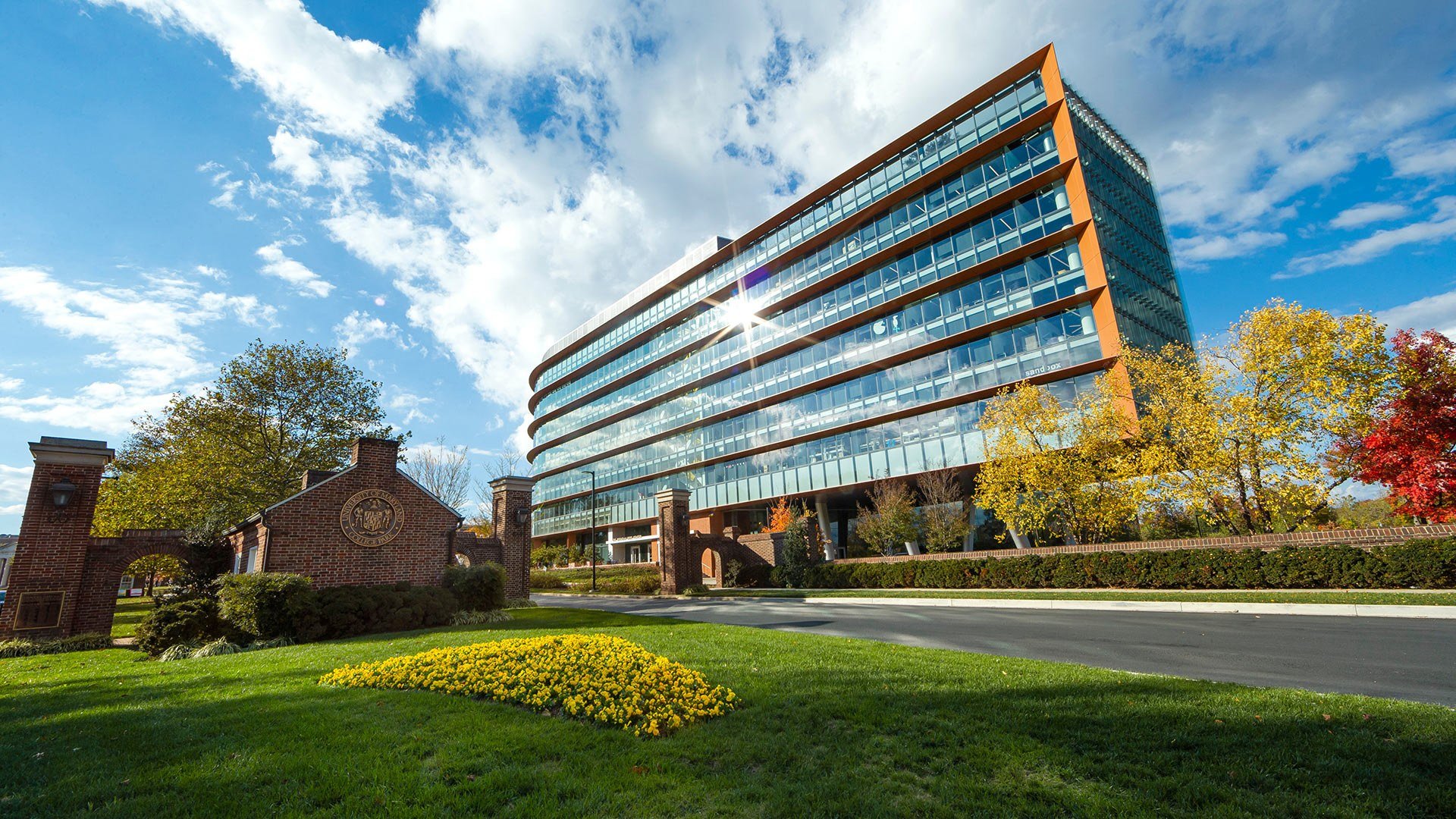
Ge Gao
Assistant Professor of Information
University of Maryland
Area of Expertise: Human-Centered Computing
Ge Gao is an assistant professor in the College of Information with an appointment in the University of Maryland Institute for Advanced Computer Studies. Her research interests lie in the field of human-computer interaction with specific focuses on computer-supported cooperative work, computer-mediated communication, and human-centered computing. Gao draws on quantitative and qualitative methods, including lab experiments, surveys, in-situ sensing and ethnography to study the joint effects of technology use and diversity on teamwork.
-
Yongle Zhang, Dennis Asamoah Owusu, Marine Carpuat, Ge Gao. Facilitating Global Team Meetings Between Language-Based Subgroups: When and How Can Machine Translation Help? Proceedings of ACM Human Computer Interaction 6(CSCW1): 90:1-90:26 (2022).
Abstract: Global teams frequently consist of language-based subgroups who put together complementary information to achieve common goals. Previous research outlines a two-step work communication flow in these teams. There are team meetings using a required common language (i.e., English); in preparation for those meetings, people have subgroup conversations in their native languages. Work communication at team meetings is often less effective than in subgroup conversations. In the current study, we investigate the idea of leveraging machine translation (MT) to facilitate global team meetings. We hypothesize that exchanging subgroup conversation logs before a team meeting offers contextual information that benefits teamwork at the meeting. MT can translate these logs, which enables comprehension at a low cost. To test our hypothesis, we conducted a between-subjects experiment where twenty quartets of participants performed a personnel selection task. Each quartet included two English native speakers (NS) and two non-native speakers (NNS) whose native language was Mandarin. All participants began the task with subgroup conversations in their native languages, then proceeded to team meetings in English. We manipulated the exchange of subgroup conversation logs prior to team meetings: with MT-mediated exchanges versus without. Analysis of participants’ subjective experience, task performance, and depth of discussions as reflected through their conversational moves jointly indicates that team meeting quality improved when there were MT-mediated exchanges of subgroup conversation logs as opposed to no exchanges. We conclude with reflections on when and how MT could be applied to enhance global teamwork across a language barrier.
-
Xu, W., Carpuat, M., & Gao, G. (2021). A Non-Autoregressive Edit-Based Approach to Controllable Text Simplification. Findings of the Association for Computational Linguistics: ACL-IJCNLP 2021, 3758–3768.
Abstract: We introduce a new approach for the task of Controllable Text Simplification, where systems rewrite a complex English sentence so that it can be understood by readers at different grade levels in the US K-12 system. It uses a non-autoregressive model to iteratively edit an input sequence and incorporates lexical complexity information seamlessly into the refinement process to generate simplifications that better match the desired output complexity than strong autoregressive baselines. Analysis shows that our model’s local edit operations are combined to achieve more complex simplification operations such as content deletion and paraphrasing, as well as sentence splitting.