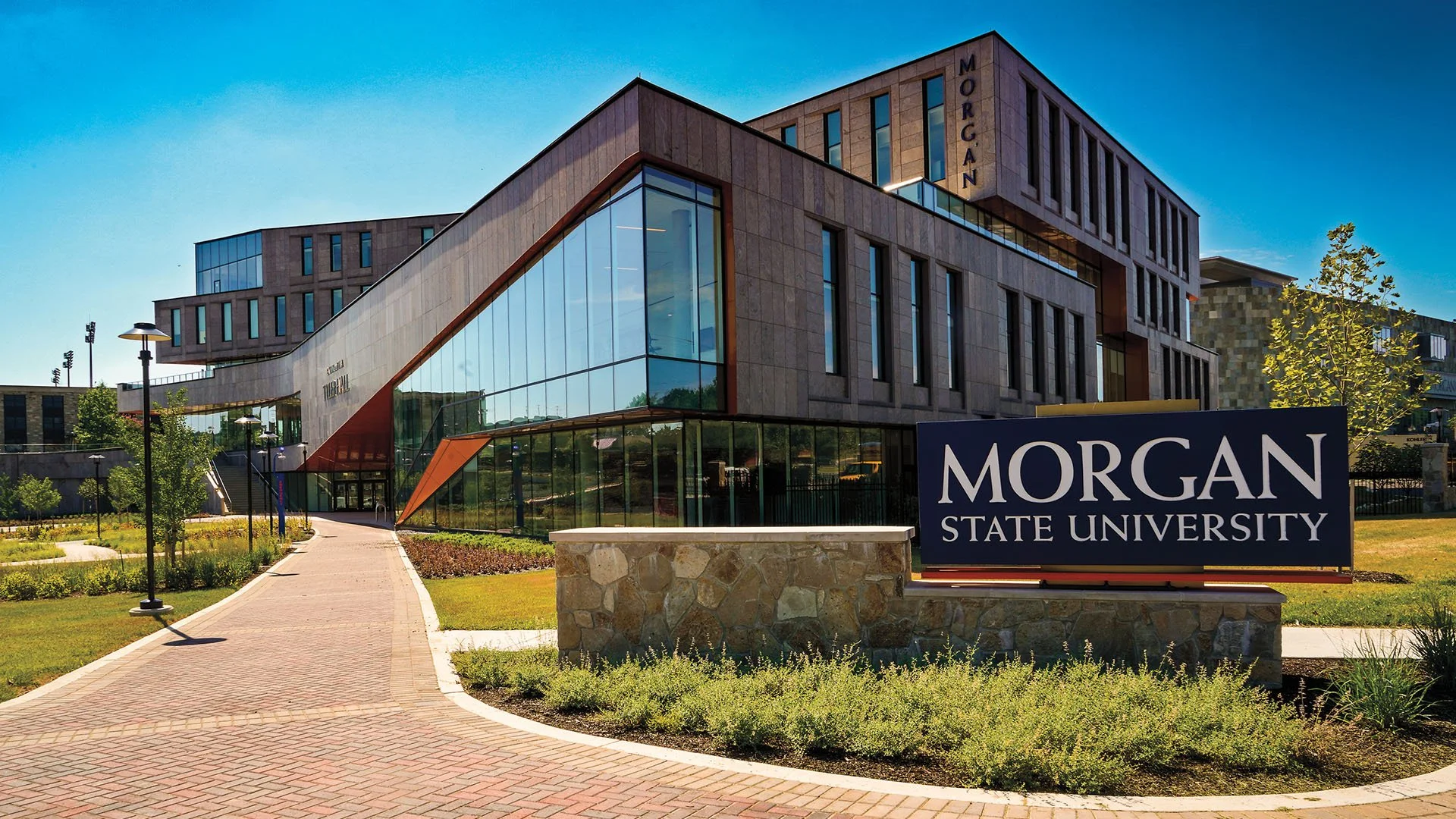
Assistant Professor of Computer Science
Monireh Dabaghchian
Morgan State University
Areas of Expertise: Wireless Communications and Network Security
Monireh Dabaghchian is an assistant professor of computer science at Morgan State University. Her research lies at the intersection of cybersecurity and machine learning. Dabaghchian studies and analyzes threats, vulnerabilities and risks present in IoT devices such as civilian unmanned aerial vehicles (UAV), smart cars, and intelligent vehicle platooning in autonomous vehicles.
-
Alipour-Fanid, A., Dabaghchian, M., Arora, R., & Zeng, K. (2022). Multiuser Scheduling in Centralized Cognitive Radio Networks: A Multi-Armed Bandit Approach. IEEE Transactions on Cognitive Communications and Networking, 8(2), 1074-1092.
Abstract: In wireless communication networks, the network provider serves certain licensed primary users who pay for a dedicated use of the frequency channels. However, not all the channels are occupied by the primary users at all times. For efficient spectrum utilization, in centralized cognitive radio networks (CRNs), a cognitive base station (CBS) dynamically identifies the spectrum holes and allocates the frequency channels to the on-demand unlicensed secondary users known as cognitive radios (CRs). Although existing literature has developed various dynamic spectrum access mechanisms for CBS, there is still a dearth of studies due to the wide range of assumptions made in the solutions. Most of the existing works study the CBS scheduling problem scheme by adopting optimization-based methods and rely on the prior knowledge of the network parameters such as primary users’ activity. Moreover, the impact of channel switching costs on the network throughput has not been well studied. In this paper, we aim to maximize the CRNs total throughput, and we formulate the CBS scheduling problem as a non-stochastic (i.e., adversarial) combinatorial multi-armed bandit problem with semi-bandit feedback and arm switching costs. We propose two novel online learning algorithms for CBS scheduling with and without channel switching costs, where their regret performances are proved sublinear order-optimal in time as T1/2 and T2/3, respectively, offering throughput-optimal scheduling for CRNs. Experiments on the synthetic and real-world spectrum measurement data complement and validate our theoretical findings.
-
Alipour-Fanid, A., Dabaghchian, M., Wang, N., Jiao, L., & Zeng, K. (2021). Online-Learning-Based Defense Against Jamming Attacks in Multichannel Wireless CPS. IEEE Internet of Things Journal, 8(17), 13278-13292.
Abstract: We study security of remote state estimation in wireless cyber-physical systems (CPS) where a sensor sends its measurements to the remote state estimator over a multichannel wireless link in presence of a jamming attacker. Most of the existing works study the sensor's defense scheme by adopting optimization-based methods and rely on the prior knowledge of the attacker's attack policy. To relax this constraint, we propose a novel online-learning-based policy called joint channel and power selection (J-CAP) for the sensor to dynamically choose transmission channel and power. The proposed method assumes no prior knowledge of the attacker's attack policy, nor of the channel state information. J-CAP jointly optimizes sensor's channel selection and power consumption, and guarantees the estimator's asymptotic stability. We theoretically prove that J-CAP achieves a sublinear learning regret bound. We also show J-CAP's optimality by deriving and matching its regret lower and upper bound orders. Compared with the solution that directly applies the baseline solution, J-CAP improves the regret upper bound by a factor of √{K+L}, where K and L denote the number of channels and number of power levels, respectively. Numerical evaluations validate the analytical results under various CPS parameters, and compare the J-CAP's performance with the state-of-the-art solutions.
-
A. Alipour-Fanid, M. Dabaghchian, N. Wang, P. Wang, L. Zhao and K. Zeng, Machine Learning-Based Delay-Aware UAV Detection Over Encrypted Wi-Fi Traffic, 2019 IEEE Conference on Communications and Network Security (CNS), Washington, DC, USA, 2019, pp. 1-7, doi: 10.1109/CNS.2019.8802721.
Abstract: In this paper, we propose a machine learning-based framework for fast UAV (unmanned aerial vehicle)detection and identification over encrypted Wi-Fi traffic. It is motivated by the observation that many consumer UAVs use Wi-Fi links for control and video streaming. The proposed framework extracts statistical features derived only from packet size and inter-arrival time of encrypted Wi-Fi traffic, and can quickly identify UAV types. In order to reduce the online identification time, our framework adopts a re-weighted ℓ 1 -norm regularization, which considers the number of samples and computation cost of different features. This framework jointly optimizes feature selection and prediction performance in a unified objective function. To tackle the packet interarrival time uncertainty when optimizing the trade-off between the identification accuracy and delay, we utilize maximum likelihood estimation (MLE)method to estimate the packet inter-arrival time. We collect a large number of real-world Wi-Fi data traffic of four types of consumer UAVs and conduct extensive evaluation on the performance of our proposed method. Evaluation results show that our proposed method can identify the tested UAVs within 0.15-0.35s with high accuracy of 85.7-95.2%.
-
M. Dabaghchian, A. Alipour-Fanid, K. Zeng, Q. Wang and P. Auer, “Online Learning With Randomized Feedback Graphs for Optimal PUE Attacks in Cognitive Radio Networks," in IEEE/ACM Transactions on Networking, vol. 26, no. 5, pp. 2268-2281, Oct. 2018, doi: 10.1109/TNET.2018.2868166.
Abstract: In a cognitive radio network, a secondary user learns the spectrum environment and dynamically accesses the channel, where the primary user is inactive. At the same time, a primary user emulation (PUE) attacker can send falsified primary user signals and prevent the secondary user from utilizing the available channel. The best attacking strategies that an attacker can apply have not been well studied. In this paper, for the first time, we study optimal PUE attack strategies by formulating an online learning problem, where the attacker needs to dynamically decide the attacking channel in each time slot based on its attacking experience. The challenge in our problem is that since the PUE attack happens in the spectrum sensing phase, the attacker cannot observe the reward on the attacked channel. To address this challenge, we utilize the attacker's observation capability. We propose online learning-based attacking strategies based on the attacker's observation capabilities. Through our analysis, we show that with no observation within the attacking slot, the attacker loses on the regret order, and with the observation of at least one channel, there is a significant improvement on the attacking performance. Observation of multiple channels does not give additional benefit to the attacker (only a constant scaling) though it gives insight on the number of observations required to achieve the minimum constant factor. Our proposed algorithms are optimal in the sense that their regret upper bounds match their corresponding regret lower bounds. We show consistency between simulation and analytical results under various system parameters.
-
Dabaghchian, M., Liu, S., Alipour-Fanid, A., Zeng, K., Li, X., & Chen, Y. (2016). Intelligence Measure of Cognitive Radios with Learning Capabilities. arXiv preprint, December 2017.
Abstract: Cognitive radio (CR) is considered as a key enabling technology for dynamic spectrum access to improve spectrum efficiency. Although the CR concept was invented with the core idea of realizing cognition, the research on measuring CR cognitive capabilities and intelligence is largely open. Deriving the intelligence measure of CR not only can lead to the development of new CR technologies, but also makes it possible to better configure the networks by integrating CRs with different cognitive capabilities. In this paper, for the first time, we propose a data-driven methodology to quantitatively measure the intelligence factors of the CR with learning capabilities. The basic idea of our methodology is to run various tests on the CR in different spectrum environments under different settings and obtain various performance data on different metrics. Then we apply factor analysis on the performance data to identify and quantize the intelligence factors and cognitive capabilities of the CR. More specifically, we present a case study consisting of 144 different types of CRs. The CRs are different in terms of learning-based dynamic spectrum access strategies, number of sensors, sensing accuracy, processing speed, and algorithmic complexity. Five intelligence factors are identified for the CRs through our data analysis. We show that these factors comply well with the nature of the tested CRs, which validates the proposed intelligence measure methodology.