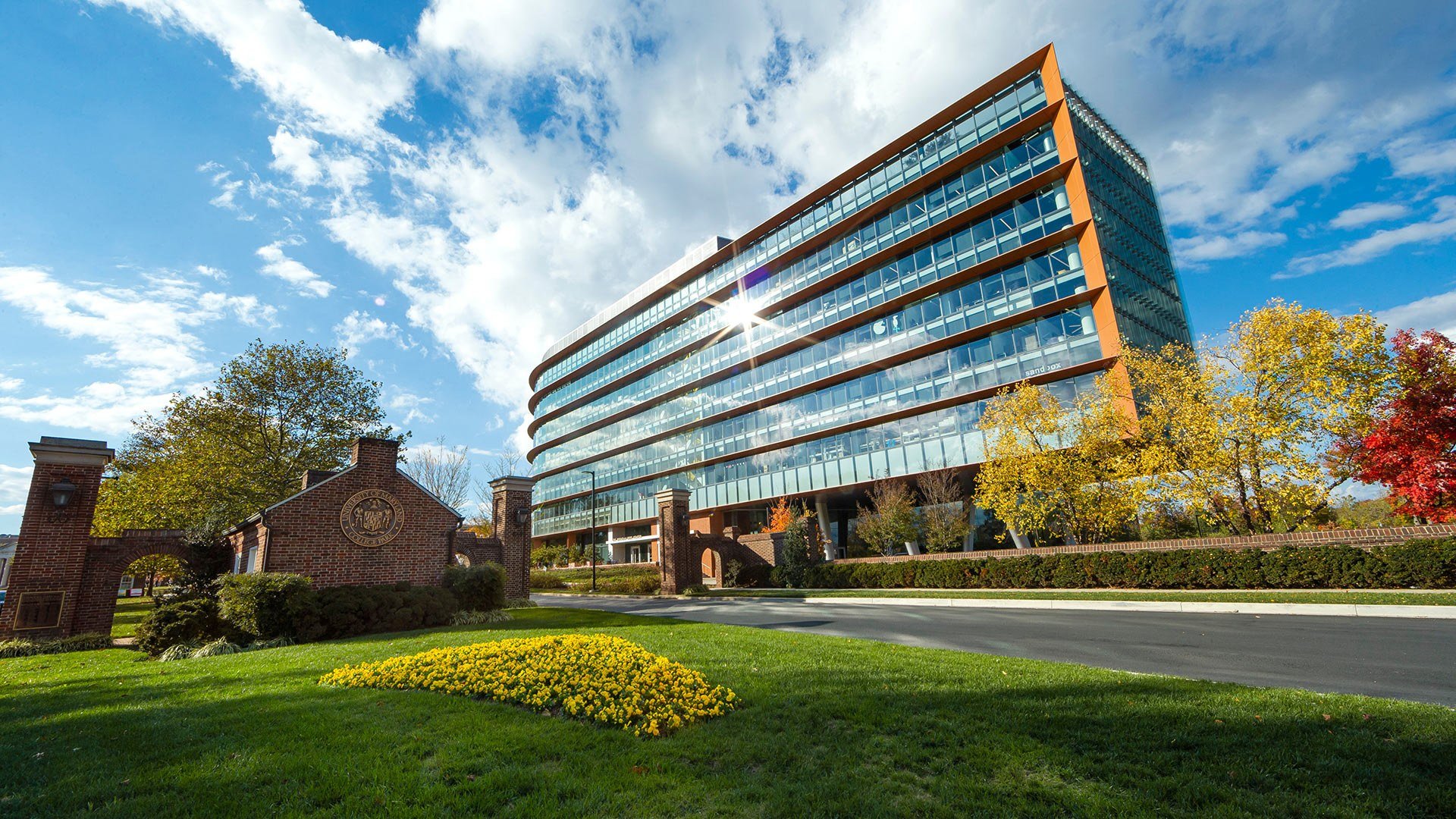
Giovanni Luca Ciampaglia
Assistant Professor, College of Information
University of Maryland
Areas of Expertise: Algorithmic Transparency, Recommendation Algorithms, & Information Integrity
Giovanni Luca Ciampaglia is an assistant professor in the College of Information at the University of Maryland. He is interested in problems originating from the interplay between people and computer systems in the determinants of information quality in cyberspace, and how information propagates across social networks, with application to the integrity of information in cyberspace, and the trustworthiness and reliability of social computing systems.
-
Bhadani, S., Yamaya, S., Flammini, A., Menczer, F., Ciampaglia, G. L., & Nyhan, B. (2022). Political Audience Diversity And News Reliability In Algorithmic Ranking. Nature Human Behaviour, 6(4), Article 4.
Abstract: Newsfeed algorithms frequently amplify misinformation and other low-quality content. How can social media platforms more effectively promote reliable information? Existing approaches are difficult to scale and vulnerable to manipulation. In this paper, we propose using the political diversity of a website’s audience as a quality signal. Using news source reliability ratings from domain experts and web browsing data from a diverse sample of 6,890 US residents, we first show that websites with more extreme and less politically diverse audiences have lower journalistic standards. We then incorporate audience diversity into a standard collaborative filtering framework and show that our improved algorithm increases the trustworthiness of websites suggested to users—especially those who most frequently consume misinformation—while keeping recommendations relevant. These findings suggest that partisan audience diversity is a valuable signal of higher journalistic standards that should be incorporated into algorithmic ranking decisions.
-
Ciampaglia, G. L., Flammini, A., & Menczer, F. (2015). The production of information in the attention economy. Scientific Reports, 5(1), Article 1.
Abstract: Online traces of human activity offer novel opportunities to study the dynamics of complex knowledge exchange networks, in particular how emergent patterns of collective attention determine what new information is generated and consumed. Can we measure the relationship between demand and supply for new information about a topic? We propose a normalization method to compare attention bursts statistics across topics with heterogeneous distribution of attention. Through analysis of a massive dataset on traffic to Wikipedia, we find that the production of new knowledge is associated to significant shifts of collective attention, which we take as proxy for its demand. This is consistent with a scenario in which allocation of attention toward a topic stimulates the demand for information about it and in turn the supply of further novel information. However, attention spikes only for a limited time span, during which new content has higher chances of receiving traffic, compared to content created later or earlier on. Our attempt to quantify demand and supply of information and our finding about their temporal ordering, may lead to the development of the fundamental laws of the attention economy and to a better understanding of social exchange of knowledge information networks.
-
Ciampaglia, G. L., Nematzadeh, A., Menczer, F., & Flammini, A. (2018). How Algorithmic Popularity Bias Hinders or Promotes Quality. Scientific Reports, 8(1), Article 1.
Abstract: Algorithms that favor popular items are used to help us select among many choices, from top-ranked search engine results to highly-cited scientific papers. The goal of these algorithms is to identify high-quality items such as reliable news, credible information sources, and important discoveries–in short, high-quality content should rank at the top. Prior work has shown that choosing what is popular may amplify random fluctuations and lead to sub-optimal rankings. Nonetheless, it is often assumed that recommending what is popular will help high-quality content “bubble up” in practice. Here we identify the conditions in which popularity may be a viable proxy for quality content by studying a simple model of a cultural market endowed with an intrinsic notion of quality. A parameter representing the cognitive cost of exploration controls the trade-off between quality and popularity. Below and above a critical exploration cost, popularity bias is more likely to hinder quality. But we find a narrow intermediate regime of user attention where an optimal balance exists: choosing what is popular can help promote high-quality items to the top. These findings clarify the effects of algorithmic popularity bias on quality outcomes, and may inform the design of more principled mechanisms for techno-social cultural markets.
-
Sasahara, K., Chen, W., Peng, H., Ciampaglia, G. L., Flammini, A., & Menczer, F. (2021). Social Influence And Unfollowing Accelerate The Emergence Of Echo Chambers. Journal of Computational Social Science, 4(1), 381–402.
Abstract: While social media make it easy to connect with and access information from anyone, they also facilitate basic influence and unfriending mechanisms that may lead to segregated and polarized clusters known as “echo chambers.” Here we study the conditions in which such echo chambers emerge by introducing a simple model of information sharing in online social networks with the two ingredients of influence and unfriending. Users can change both their opinions and social connections based on the information to which they are exposed through sharing. The model dynamics show that even with minimal amounts of influence and unfriending, the social network rapidly devolves into segregated, homogeneous communities. These predictions are consistent with empirical data from Twitter. Although our findings suggest that echo chambers are somewhat inevitable given the mechanisms at play in online social media, they also provide insights into possible mitigation strategies.
-
Shao, C., Ciampaglia, G. L., Varol, O., Yang, K.-C., Flammini, A., & Menczer, F. (2018). The Spread of Low-Credibility Content by Social Bots. Nature Communications, 9(1), Article 1.
Abstract: The massive spread of digital misinformation has been identified as a major threat to democracies. Communication, cognitive, social, and computer scientists are studying the complex causes for the viral diffusion of misinformation, while online platforms are beginning to deploy countermeasures. Little systematic, data-based evidence has been published to guide these efforts. Here we analyze 14 million messages spreading 400 thousand articles on Twitter during ten months in 2016 and 2017. We find evidence that social bots played a disproportionate role in spreading articles from low-credibility sources. Bots amplify such content in the early spreading moments, before an article goes viral. They also target users with many followers through replies and mentions. Humans are vulnerable to this manipulation, resharing content posted by bots. Successful low-credibility sources are heavily supported by social bots. These results suggest that curbing social bots may be an effective strategy for mitigating the spread of online misinformation.