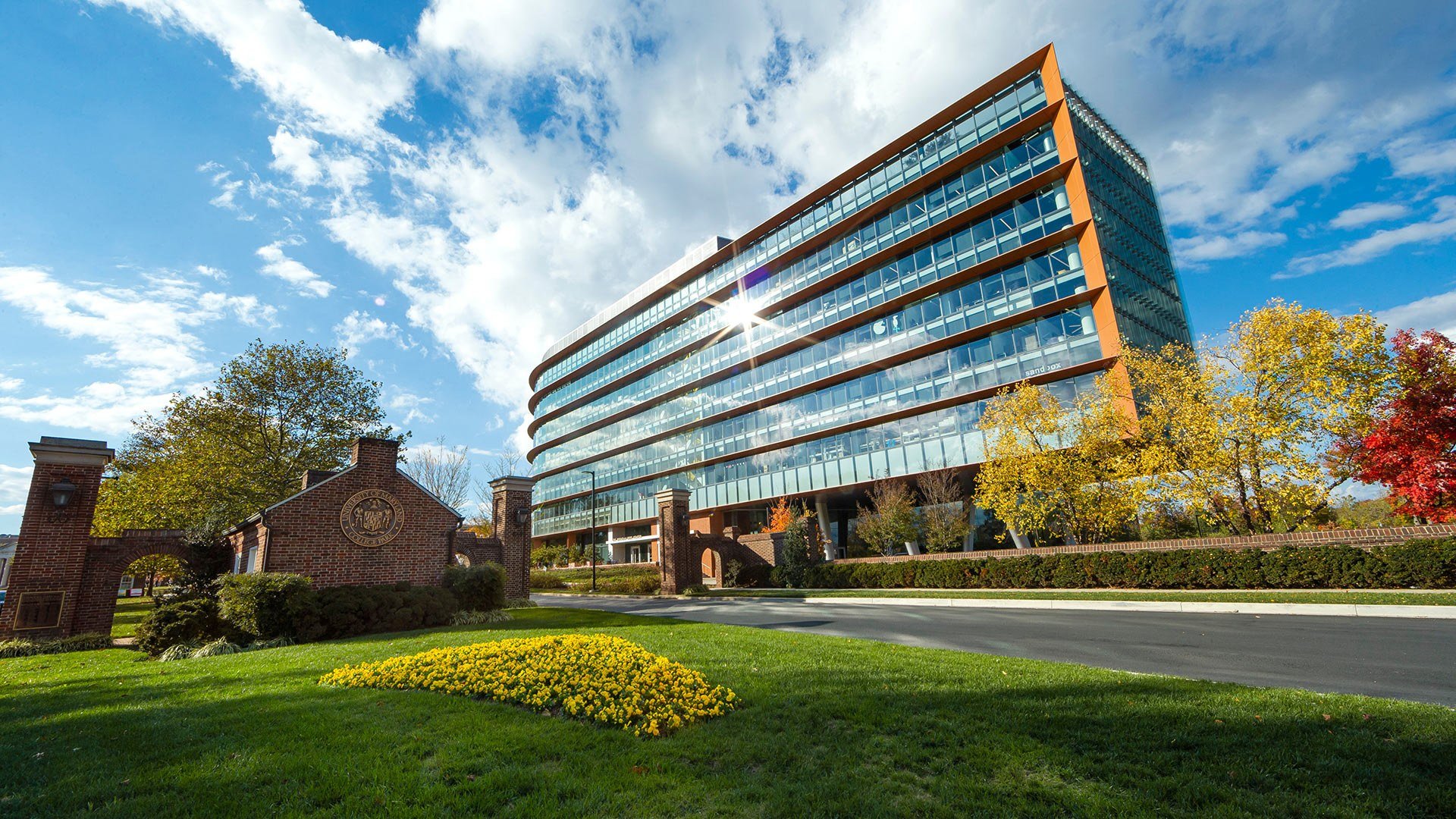
Marine Carpuat
Associate Professor of Computer Science
University of Maryland
Areas of Expertise: Trustworthy Natural Language Processing (NLP)
Marine Carpuat is an associate professor of computer science with an appointment in the University of Maryland Institute for Advanced Computer Studies where she is also a member of the Computational Linguistics and Information Processing Laboratory. Carpuat’s research focuses on NLP and machine translation to develop technologies that help people communicate across languages.
-
Weijia Xu, Sweta Agrawal, Eleftheria Briakou, Marianna J. Martindale, Marine Carpuat. Understanding and Detecting Hallucinations in Neural Machine Translation via Model Introspection. Transactions of the Association for Computational Linguistics (2023) 11: 546–564.
Abstract: Neural sequence generation models are known to “hallucinate”, by producing outputs that are unrelated to the source text. These hallucinations are potentially harmful, yet it remains unclear in what conditions they arise and how to mitigate their impact. In this work, we first identify internal model symptoms of hallucinations by analyzing the relative token contributions to the generation in contrastive hallucinated vs. non-hallucinated outputs generated via source perturbations. We then show that these symptoms are reliable indicators of natural hallucinations, by using them to design a lightweight hallucination detector which outperforms both model-free baselines and strong classifiers based on quality estimation or large pre-trained models on manually annotated English-Chinese and German-English translation test beds.
-
Yongle Zhang, Dennis Asamoah Owusu, Marine Carpuat, Ge Gao. Facilitating Global Team Meetings Between Language-Based Subgroups: When and How Can Machine Translation Help? Proceedings of ACM Human Computer Interaction 6(CSCW1): 90:1-90:26 (2022).
Abstract: Global teams frequently consist of language-based subgroups who put together complementary information to achieve common goals. Previous research outlines a two-step work communication flow in these teams. There are team meetings using a required common language (i.e., English); in preparation for those meetings, people have subgroup conversations in their native languages. Work communication at team meetings is often less effective than in subgroup conversations. In the current study, we investigate the idea of leveraging machine translation (MT) to facilitate global team meetings. We hypothesize that exchanging subgroup conversation logs before a team meeting offers contextual information that benefits teamwork at the meeting. MT can translate these logs, which enables comprehension at a low cost. To test our hypothesis, we conducted a between-subjects experiment where twenty quartets of participants performed a personnel selection task. Each quartet included two English native speakers (NS) and two non-native speakers (NNS) whose native language was Mandarin. All participants began the task with subgroup conversations in their native languages, then proceeded to team meetings in English. We manipulated the exchange of subgroup conversation logs prior to team meetings: with MT-mediated exchanges versus without. Analysis of participants’ subjective experience, task performance, and depth of discussions as reflected through their conversational moves jointly indicates that team meeting quality improved when there were MT-mediated exchanges of subgroup conversation logs as opposed to no exchanges. We conclude with reflections on when and how MT could be applied to enhance global teamwork across a language barrier.
-
Xu, W., Carpuat, M., & Gao, G. (2021). A Non-Autoregressive Edit-Based Approach to Controllable Text Simplification. Findings of the Association for Computational Linguistics: ACL-IJCNLP 2021, 3758–3768.
Abstract: We introduce a new approach for the task of Controllable Text Simplification, where systems rewrite a complex English sentence so that it can be understood by readers at different grade levels in the US K-12 system. It uses a non-autoregressive model to iteratively edit an input sequence and incorporates lexical complexity information seamlessly into the refinement process to generate simplifications that better match the desired output complexity than strong autoregressive baselines. Analysis shows that our model’s local edit operations are combined to achieve more complex simplification operations such as content deletion and paraphrasing, as well as sentence splitting.
-
Niu, X., Rao, S., & Carpuat, M. (2018). Multi-Task Neural Models for Translating Between Styles Within and Across Languages. Proceedings of the 27th International Conference on Computational Linguistics, 1008–1021.
Abstract: Generating natural language requires conveying content in an appropriate style. We explore two related tasks on generating text of varying formality: monolingual formality transfer and formality-sensitive machine translation. We propose to solve these tasks jointly using multi-task learning, and show that our models achieve state-of-the-art performance for formality transfer and are able to perform formality-sensitive translation without being explicitly trained on style-annotated translation examples.
-
Martindale, M., & Carpuat, M. (2018). Fluency Over Adequacy: A Pilot Study in Measuring User Trust in Imperfect MT. In Proceedings of AMTA 2018, vol. 1: MT Research Track, Boston, March 17 - 21, 2018, pp. 13-25.
Abstract: Although measuring intrinsic quality has been a key factor in the advancement of Machine Translation (MT), successfully deploying MT requires considering not just intrinsic quality but also the user experience, including aspects such as trust. This work introduces a method of studying how users modulate their trust in an MT system after seeing errorful (disfluent or inadequate) output amidst good (fluent and adequate) output. We conduct a survey to determine how users respond to good translations compared to translations that are either adequate but not fluent, or fluent but not adequate. In this pilot study, users responded strongly to disfluent translations, but were, surprisingly, much less concerned with adequacy.