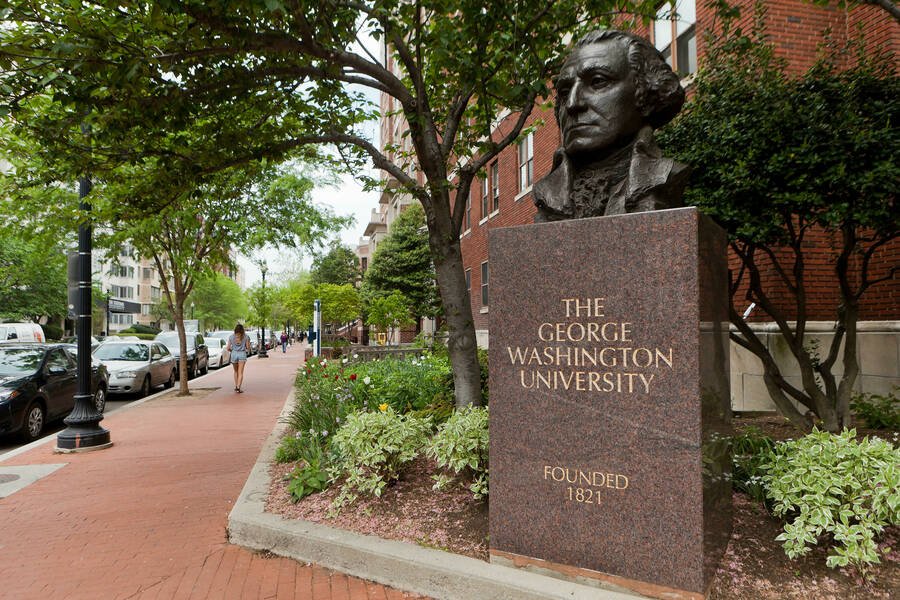
David Broniatowski, Co-PI
Professor of Engineering Management and Systems Engineering
George Washington University Site Lead
David Broniatowski is a professor of engineering management and systems engineering at GW. He conducts research in decision-making under risk, group decision-making, system architecture, and behavioral epidemiology. Broniatowski uses a wide-range of techniques including formal mathematical modeling, experimental design, automated text analysis and natural language processing, social and technical network analysis, and big data. As a co-PI of TRAILS, he directs research on effectively evaluating trust of AI systems, including how people make sense of them, and the degree to which levels of reliability, fairness, transparency and accountability translate to the appropriate levels of trust.
Area of Expertise: AI Evaluation
-
David A. Broniatowski et al. The Efficacy of Facebook’s Vaccine Misinformation Policies and Architecture During the Covid-19 Pandemic. Sci. Adv. 9, eadh2132 (2023).
Abstract: Online misinformation promotes distrust in science, undermines public health, and may drive civil unrest. During the coronavirus disease 2019 pandemic, Facebook—the world’s largest social media company—began to remove vaccine misinformation as a matter of policy. We evaluated the efficacy of these policies using a comparative interrupted time-series design. We found that Facebook removed some anti-vaccine content, but we did not observe decreases in overall engagement with anti-vaccine content. Pro-vaccine content was also removed, and anti-vaccine content became more misinformative, more politically polarized, and more likely to be seen in users’ newsfeeds. We explain these findings as a consequence of Facebook’s system architecture, which provides substantial flexibility to motivated users who wish to disseminate misinformation through multiple channels. Facebook’s architecture may therefore afford antivaccine content producers several means to circumvent the intent of misinformation removal policies.
-
Broniatowski, D. (2021). Psychological Foundations of Explainability and Interpretability in Artificial Intelligence, NIST Interagency/Internal Report (NISTIR), National Institute of Standards and Technology, Gaithersburg, MD.
Abstract: In this paper, we make the case that interpretability and explainability are distinct requirements for machine learning systems. To make this case, we provide an overview of the literature in experimental psychology pertaining to interpretation (especially of numerical stimuli) and comprehension. We find that interpretation refers to the ability to contextualize a model’s output in a manner that relates it to the system’s designed functional purpose, and the goals, values, and preferences of end users. In contrast, explanation refers to the ability to accurately describe the mechanism, or implementation, that led to an algorithm’s output, often so that the algorithm can be improved in some way. Beyond these definitions, our review shows that humans differ from one another in systematic ways, that affect the extent to which they prefer to make decisions based on detailed explanations versus less precise interpretations. These individual differences, such as personality traits and skills, are associated with their abilities to derive meaningful interpretations from precise explanations of model output. This implies that system output should be tailored to different types of users.
-
Broniatowski, D. A. (2019). Communicating Meaning in the Intelligence Enterprise. Policy Insights from the Behavioral and Brain Sciences, 6(1), 38–46.
Abstract: Intelligence community experts face challenges communicating the results of analysis products to policy makers. Given the high-stakes nature of intelligence analyses, the consequences of misinformation may be dire, potentially leading to costly, ill-informed policies or lasting damage to national security. Much is known regarding how to effectively communicate complex analysis products to policy makers possessing different sources of expertise. Fuzzy-Trace Theory, an empirically-validated psychological account of how decision makers derive meaning from complex stimuli, emphasizes the importance of communicating the essential bottom-line of an analysis (“gist”), in parallel with precise details (“verbatim”). Verbatim details can be prone to misinterpretation when presented out of context. Several examples from intelligence analyses and laboratory studies are discussed, with implications for integrating knowledge from multiple sources of expertise, communicating complex technical information to nontechnical recipients, and identifying and training effective communicators. Collaboration between the academic and intelligence communities would facilitate new insights and scientifically grounded implementation of findings.
-
Marti, D, Broniatowski, DA. Does Gist Drive NASA Experts’ Design Decisions? Systems Engineering. 2020; 23: 460– 479.
Abstract: As engineers retire from practice, they must transfer their expertise to new recruits. Typically, this is accomplished using decision-support systems that communicate precise probabilities. However, Fuzzy-Trace Theory (FTT) predicts that most experts prefer to rely on “gist” representations of risk over “verbatim” representations. We conducted a survey of 41 NASA employees (whose mathematical abilities are a prerequisite for their jobs) and 233 non-experts. We tested whether experts designing space missions under the micrometeoroid and orbital debris (MMOD) impact – rely more on qualitative or quantitative risk representations. We tested three hypotheses: gist and verbatim representations of MMOD risk are distinct for both experts and non-experts; gist representations are more predictive of decisions than are verbatim representations; and providing non-experts with a bottom-line meaning change their gists more than verbatim information does. Results support FTT's predictions: gist and verbatim representations were distinct, and gist representations were associated with decisions for both experts and non-experts. We did not observe an association between quantitative risk estimates and decisions for either experts or non-experts. We observed that exposing a non-expert to an expert's gist modified that non-expert's gist yet exposing quantitative risk information did not. Implications for expertise transfer are discussed.
-
Broniatowski, D. A., & Tucker, C. (2017). Assessing Causal Claims About Complex Engineered Systems With Quantitative Data: Internal, External, And Construct Validity. Systems Engineering, 20(6), 483–496.
Abstract: Engineers seek to design systems that will produce an intended change in the state of the world. How are we to know if a system will behave as intended? This article addresses ways that this question can be answered. Specifically, we focus on three types of research validity: (1) internal validity, or whether an observed association between two variables can be attributed to a causal link between them; (2) external validity, or whether a causal link generalizes across contexts; and (3) construct validity, or whether a specific set of metrics corresponds to what they are intended to measure. In each case, we discuss techniques that may be used to establish the corresponding type of validity: namely, quasi-experimental design, replication, and establishment of convergent-discriminant validity and reliability. These techniques typically require access to data, which has historically been limited for research on complex engineered systems. This is likely to change in the era of “big data.” Thus, we discuss the continued utility of these validity concepts in the face of advances in machine learning and big data as they pertain to complex engineered sociotechnical systems. Next, we discuss relationships between these validity concepts and other prominent approaches to evaluating research in the field. Finally, we propose a set of criteria by which one may evaluate research utilizing quantitative observation to test causal theory in the field of complex engineered systems.
-
Szajnfarber, Z., & Broniatowski, D. A. (2020). Research Methods for Supporting Engineering Systems Design. In A. Maier, J. Oehmen, & P. E. Vermaas (Eds.), Handbook of Engineering Systems Design (pp. 1–26).
Abstract: Engineering systems, with their technical and social, cyber, and physical components interacting, are best understood when studied from multiple methodological lenses simultaneously. However, since different methodological paradigms have grown up in different disciplinary traditions, it is often challenging for researchers to draw on insights across them. In this chapter, we review four methodological paradigms of research done on engineering systems: 1. Quantitative observational research, including inferential statistics and machine learning 2. Qualitative observational research, which infer causal mechanisms based on deep contextual understanding 3. In vivo experiments and quasi-experiments, which manipulate theoretically motivated variables in more or less controlled settings to establish causality 4. In silico experiments, which deductively explore the consequences of a mathematical representation of reality